Note
-
Download Jupyter notebook:
https://docs.doubleml.org/stable/examples/did/py_panel.ipynb.
Python: Panel Data with Multiple Time Periods#
In this example, a detailed guide on Difference-in-Differences with multiple time periods using the DoubleML-package. The implementation is based on Callaway and Sant’Anna(2021).
The notebook requires the following packages:
[1]:
import seaborn as sns
import matplotlib.pyplot as plt
import pandas as pd
import numpy as np
from lightgbm import LGBMRegressor, LGBMClassifier
from sklearn.linear_model import LinearRegression, LogisticRegression
from doubleml.did import DoubleMLDIDMulti
from doubleml.data import DoubleMLPanelData
from doubleml.did.datasets import make_did_CS2021
Data#
We will rely on the make_did_CS2021
DGP, which is inspired by Callaway and Sant’Anna(2021) (Appendix SC) and Sant’Anna and Zhao (2020).
We will observe n_obs
units over n_periods
. Remark that the dataframe includes observations of the potential outcomes y0
and y1
, such that we can use oracle estimates as comparisons.
[2]:
n_obs = 5000
n_periods = 6
df = make_did_CS2021(n_obs, dgp_type=4, n_periods=n_periods, n_pre_treat_periods=3, time_type="datetime")
df["ite"] = df["y1"] - df["y0"]
print(df.shape)
df.head()
(30000, 11)
[2]:
id | y | y0 | y1 | d | t | Z1 | Z2 | Z3 | Z4 | ite | |
---|---|---|---|---|---|---|---|---|---|---|---|
0 | 0 | 217.520035 | 217.520035 | 216.002945 | 2025-04-01 | 2025-01-01 | 1.592538 | 0.168945 | 0.417931 | -0.167139 | -1.517090 |
1 | 0 | 226.589217 | 226.589217 | 224.203601 | 2025-04-01 | 2025-02-01 | 1.592538 | 0.168945 | 0.417931 | -0.167139 | -2.385616 |
2 | 0 | 233.790397 | 233.790397 | 234.656805 | 2025-04-01 | 2025-03-01 | 1.592538 | 0.168945 | 0.417931 | -0.167139 | 0.866408 |
3 | 0 | 239.848096 | 241.437147 | 239.848096 | 2025-04-01 | 2025-04-01 | 1.592538 | 0.168945 | 0.417931 | -0.167139 | -1.589051 |
4 | 0 | 250.239014 | 249.466407 | 250.239014 | 2025-04-01 | 2025-05-01 | 1.592538 | 0.168945 | 0.417931 | -0.167139 | 0.772607 |
Data Details#
Here, we slightly abuse the definition of the potential outcomes. :math:`Y_{i,t}(1)` corresponds to the (potential) outcome if unit :math:`i` would have received treatment at time period :math:`mathrm{g}` (where the group :math:`mathrm{g}` is drawn with probabilities based on :math:`Z`).
More specifically
where
\(f_t(Z)\) depends on pre-treatment observable covariates \(Z_1,\dots, Z_4\) and time \(t\)
\(\delta_t\) is a time fixed effect
\(\eta_i\) is a unit fixed effect
\(\epsilon_{i,t,\cdot}\) are time varying unobservables (iid. \(N(0,1)\))
\(\theta_{i,t,\mathrm{g}}\) correponds to the exposure effect of unit \(i\) based on group \(\mathrm{g}\) at time \(t\)
For the pre-treatment periods the exposure effect is set to
such that
The DoubleML Coverage Repository includes coverage simulations based on this DGP.
Data Description#
The data is a balanced panel where each unit is observed over n_periods
starting Janary 2025.
[3]:
df.groupby("t").size()
[3]:
t
2025-01-01 5000
2025-02-01 5000
2025-03-01 5000
2025-04-01 5000
2025-05-01 5000
2025-06-01 5000
dtype: int64
The treatment column d
indicates first treatment period of the corresponding unit, whereas NaT
units are never treated.
Generally, never treated units should take either on the value ``np.inf`` or ``pd.NaT`` depending on the data type (``float`` or ``datetime``).
The individual units are roughly uniformly divided between the groups, where treatment assignment depends on the pre-treatment covariates Z1
to Z4
.
[4]:
df.groupby("d", dropna=False).size()
[4]:
d
2025-04-01 7998
2025-05-01 6774
2025-06-01 7260
NaT 7968
dtype: int64
Here, the group indicates the first treated period and NaT
units are never treated. To simplify plotting and pands
[5]:
df.groupby("d", dropna=False).size()
[5]:
d
2025-04-01 7998
2025-05-01 6774
2025-06-01 7260
NaT 7968
dtype: int64
To get a better understanding of the underlying data and true effects, we will compare the unconditional averages and the true effects based on the oracle values of individual effects ite
.
[6]:
# rename for plotting
df["First Treated"] = df["d"].dt.strftime("%Y-%m").fillna("Never Treated")
# Create aggregation dictionary for means
def agg_dict(col_name):
return {
f'{col_name}_mean': (col_name, 'mean'),
f'{col_name}_lower_quantile': (col_name, lambda x: x.quantile(0.05)),
f'{col_name}_upper_quantile': (col_name, lambda x: x.quantile(0.95))
}
# Calculate means and confidence intervals
agg_dictionary = agg_dict("y") | agg_dict("ite")
agg_df = df.groupby(["t", "First Treated"]).agg(**agg_dictionary).reset_index()
agg_df.head()
[6]:
t | First Treated | y_mean | y_lower_quantile | y_upper_quantile | ite_mean | ite_lower_quantile | ite_upper_quantile | |
---|---|---|---|---|---|---|---|---|
0 | 2025-01-01 | 2025-04 | 208.647546 | 198.418840 | 218.718684 | 0.030083 | -2.341894 | 2.382756 |
1 | 2025-01-01 | 2025-05 | 210.530041 | 200.200676 | 219.943050 | -0.026408 | -2.256611 | 2.247845 |
2 | 2025-01-01 | 2025-06 | 212.272490 | 202.594733 | 222.385196 | -0.040196 | -2.261819 | 2.191859 |
3 | 2025-01-01 | Never Treated | 214.375830 | 204.411924 | 224.581491 | 0.042591 | -2.129263 | 2.332571 |
4 | 2025-02-01 | 2025-04 | 208.367984 | 188.255466 | 228.274841 | -0.027047 | -2.362394 | 2.202422 |
[7]:
def plot_data(df, col_name='y'):
"""
Create an improved plot with colorblind-friendly features
Parameters:
-----------
df : DataFrame
The dataframe containing the data
col_name : str, default='y'
Column name to plot (will use '{col_name}_mean')
"""
plt.figure(figsize=(12, 7))
n_colors = df["First Treated"].nunique()
color_palette = sns.color_palette("colorblind", n_colors=n_colors)
sns.lineplot(
data=df,
x='t',
y=f'{col_name}_mean',
hue='First Treated',
style='First Treated',
palette=color_palette,
markers=True,
dashes=True,
linewidth=2.5,
alpha=0.8
)
plt.title(f'Average Values {col_name} by Group Over Time', fontsize=16)
plt.xlabel('Time', fontsize=14)
plt.ylabel(f'Average Value {col_name}', fontsize=14)
plt.legend(title='First Treated', title_fontsize=13, fontsize=12,
frameon=True, framealpha=0.9, loc='best')
plt.grid(alpha=0.3, linestyle='-')
plt.tight_layout()
plt.show()
So let us take a look at the average values over time
[8]:
plot_data(agg_df, col_name='y')
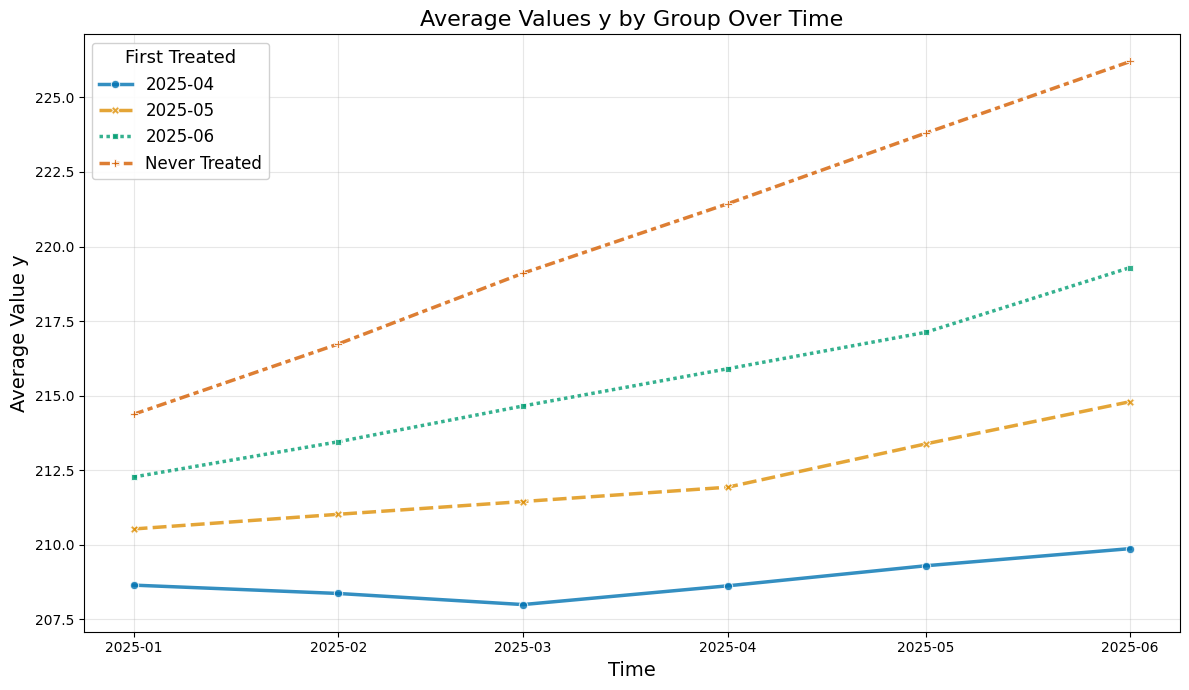
Instead the true average treatment treatment effects can be obtained by averaging (usually unobserved) the ite
values.
The true effect just equals the exposure time (in months):
[9]:
plot_data(agg_df, col_name='ite')
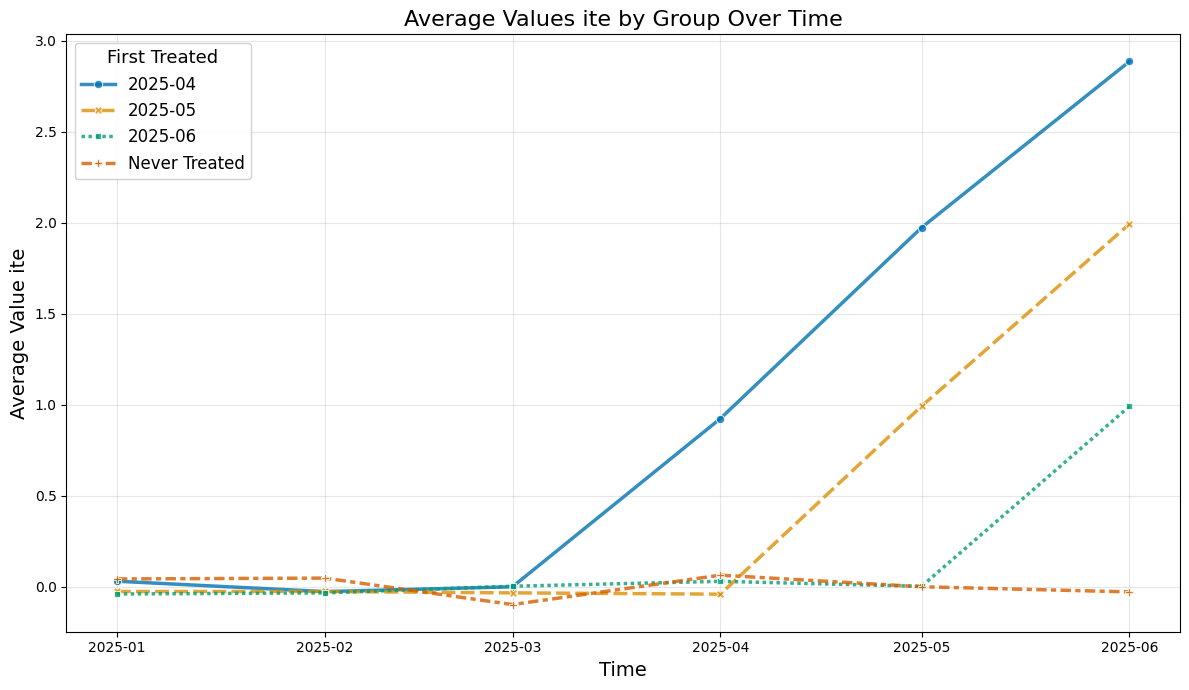
DoubleMLPanelData#
Finally, we can construct our DoubleMLPanelData
, specifying
y_col
: the outcomed_cols
: the group variable indicating the first treated period for each unitid_col
: the unique identification column for each unitt_col
: the time columnx_cols
: the additional pre-treatment controlsdatetime_unit
: unit required fordatetime
columns and plotting
[10]:
dml_data = DoubleMLPanelData(
data=df,
y_col="y",
d_cols="d",
id_col="id",
t_col="t",
x_cols=["Z1", "Z2", "Z3", "Z4"],
datetime_unit="M"
)
print(dml_data)
================== DoubleMLPanelData Object ==================
------------------ Data summary ------------------
Outcome variable: y
Treatment variable(s): ['d']
Covariates: ['Z1', 'Z2', 'Z3', 'Z4']
Instrument variable(s): None
Time variable: t
Id variable: id
No. Observations: 5000
------------------ DataFrame info ------------------
<class 'pandas.core.frame.DataFrame'>
RangeIndex: 30000 entries, 0 to 29999
Columns: 12 entries, id to First Treated
dtypes: datetime64[s](2), float64(8), int64(1), object(1)
memory usage: 2.7+ MB
ATT Estimation#
The DoubleML-package implements estimation of group-time average treatment effect via the DoubleMLDIDMulti
class (see model documentation).
Basics#
The class basically behaves like other DoubleML
classes and requires the specification of two learners (for more details on the regression elements, see score documentation).
The basic arguments of a DoubleMLDIDMulti
object include
ml_g
“outcome” regression learnerml_m
propensity Score learnercontrol_group
the control group for the parallel trend assumptiongt_combinations
combinations of \((\mathrm{g},t_\text{pre}, t_\text{eval})\)anticipation_periods
number of anticipation periods
We will construct a dict
with “default” arguments.
[11]:
default_args = {
"ml_g": LGBMRegressor(n_estimators=500, learning_rate=0.01, verbose=-1, random_state=123),
"ml_m": LGBMClassifier(n_estimators=500, learning_rate=0.01, verbose=-1, random_state=123),
"control_group": "never_treated",
"gt_combinations": "standard",
"anticipation_periods": 0,
"n_folds": 5,
"n_rep": 1,
}
The model will be estimated using the fit()
method.
[12]:
dml_obj = DoubleMLDIDMulti(dml_data, **default_args)
dml_obj.fit()
print(dml_obj)
================== DoubleMLDIDMulti Object ==================
------------------ Data summary ------------------
Outcome variable: y
Treatment variable(s): ['d']
Covariates: ['Z1', 'Z2', 'Z3', 'Z4']
Instrument variable(s): None
Time variable: t
Id variable: id
No. Observations: 5000
------------------ Score & algorithm ------------------
Score function: observational
Control group: never_treated
Anticipation periods: 0
------------------ Machine learner ------------------
Learner ml_g: LGBMRegressor(learning_rate=0.01, n_estimators=500, random_state=123,
verbose=-1)
Learner ml_m: LGBMClassifier(learning_rate=0.01, n_estimators=500, random_state=123,
verbose=-1)
Out-of-sample Performance:
Regression:
Learner ml_g0 RMSE: [[1.94152039 1.88184715 1.91629957 2.87236332 3.99291212 1.94222811
1.88439593 1.93625074 1.92448279 2.80632692 1.90447934 1.85435272
1.92050314 1.93145292 1.95743595]]
Learner ml_g1 RMSE: [[1.91908951 1.97108037 1.94177756 2.80099131 3.9070048 1.90830613
1.99120791 2.04191493 1.95593078 2.92398607 1.90963022 1.79705541
1.92075645 1.88252248 1.93271618]]
Classification:
Learner ml_m Log Loss: [[0.66389729 0.65435231 0.66535079 0.65733152 0.65807126 0.69195036
0.68704901 0.69001246 0.69948765 0.69000151 0.72486633 0.72775035
0.72290484 0.73042451 0.71721858]]
------------------ Resampling ------------------
No. folds: 5
No. repeated sample splits: 1
------------------ Fit summary ------------------
coef std err t P>|t| \
ATT(2025-04,2025-01,2025-02) -0.149130 0.125997 -1.183594 2.365739e-01
ATT(2025-04,2025-02,2025-03) -0.139825 0.104174 -1.342225 1.795230e-01
ATT(2025-04,2025-03,2025-04) 0.795353 0.105858 7.513365 5.773160e-14
ATT(2025-04,2025-03,2025-05) 1.715979 0.213360 8.042642 8.881784e-16
ATT(2025-04,2025-03,2025-06) 2.344832 0.406269 5.771623 7.851150e-09
ATT(2025-05,2025-01,2025-02) -0.071531 0.102905 -0.695121 4.869792e-01
ATT(2025-05,2025-02,2025-03) -0.112429 0.095312 -1.179584 2.381655e-01
ATT(2025-05,2025-03,2025-04) -0.006604 0.102809 -0.064238 9.487809e-01
ATT(2025-05,2025-04,2025-05) 0.939796 0.100396 9.360866 0.000000e+00
ATT(2025-05,2025-04,2025-06) 1.829721 0.150206 12.181404 0.000000e+00
ATT(2025-06,2025-01,2025-02) -0.126291 0.096670 -1.306414 1.914117e-01
ATT(2025-06,2025-02,2025-03) -0.039415 0.082845 -0.475769 6.342388e-01
ATT(2025-06,2025-03,2025-04) 0.043218 0.089757 0.481499 6.301616e-01
ATT(2025-06,2025-04,2025-05) -0.003861 0.099469 -0.038821 9.690335e-01
ATT(2025-06,2025-05,2025-06) 0.798295 0.090798 8.792034 0.000000e+00
2.5 % 97.5 %
ATT(2025-04,2025-01,2025-02) -0.396080 0.097821
ATT(2025-04,2025-02,2025-03) -0.344003 0.064353
ATT(2025-04,2025-03,2025-04) 0.587874 1.002831
ATT(2025-04,2025-03,2025-05) 1.297801 2.134157
ATT(2025-04,2025-03,2025-06) 1.548559 3.141105
ATT(2025-05,2025-01,2025-02) -0.273222 0.130159
ATT(2025-05,2025-02,2025-03) -0.299237 0.074380
ATT(2025-05,2025-03,2025-04) -0.208105 0.194897
ATT(2025-05,2025-04,2025-05) 0.743023 1.136568
ATT(2025-05,2025-04,2025-06) 1.535323 2.124120
ATT(2025-06,2025-01,2025-02) -0.315761 0.063179
ATT(2025-06,2025-02,2025-03) -0.201787 0.122957
ATT(2025-06,2025-03,2025-04) -0.132703 0.219139
ATT(2025-06,2025-04,2025-05) -0.198817 0.191094
ATT(2025-06,2025-05,2025-06) 0.620335 0.976255
The summary displays estimates of the \(ATT(g,t_\text{eval})\) effects for different combinations of \((g,t_\text{eval})\) via \(\widehat{ATT}(\mathrm{g},t_\text{pre},t_\text{eval})\), where
\(\mathrm{g}\) specifies the group
\(t_\text{pre}\) specifies the corresponding pre-treatment period
\(t_\text{eval}\) specifies the evaluation period
The choice gt_combinations="standard"
, used estimates all possible combinations of \(ATT(g,t_\text{eval})\) via \(\widehat{ATT}(\mathrm{g},t_\text{pre},t_\text{eval})\), where the standard choice is \(t_\text{pre} = \min(\mathrm{g}, t_\text{eval}) - 1\) (without anticipation).
Remark that this includes pre-tests effects if \(\mathrm{g} > t_{eval}\), e.g. \(\widehat{ATT}(g=\text{2025-04}, t_{\text{pre}}=\text{2025-01}, t_{\text{eval}}=\text{2025-02})\) which estimates the pre-trend from January to February even if the actual treatment occured in April.
As usual for the DoubleML-package, you can obtain joint confidence intervals via bootstrap.
[13]:
level = 0.95
ci = dml_obj.confint(level=level)
dml_obj.bootstrap(n_rep_boot=5000)
ci_joint = dml_obj.confint(level=level, joint=True)
ci_joint
[13]:
2.5 % | 97.5 % | |
---|---|---|
ATT(2025-04,2025-01,2025-02) | -0.513173 | 0.214913 |
ATT(2025-04,2025-02,2025-03) | -0.440815 | 0.161164 |
ATT(2025-04,2025-03,2025-04) | 0.489497 | 1.101208 |
ATT(2025-04,2025-03,2025-05) | 1.099521 | 2.332438 |
ATT(2025-04,2025-03,2025-06) | 1.171004 | 3.518660 |
ATT(2025-05,2025-01,2025-02) | -0.368854 | 0.225791 |
ATT(2025-05,2025-02,2025-03) | -0.387813 | 0.162956 |
ATT(2025-05,2025-03,2025-04) | -0.303648 | 0.290439 |
ATT(2025-05,2025-04,2025-05) | 0.649722 | 1.229869 |
ATT(2025-05,2025-04,2025-06) | 1.395733 | 2.263710 |
ATT(2025-06,2025-01,2025-02) | -0.405599 | 0.153017 |
ATT(2025-06,2025-02,2025-03) | -0.278777 | 0.199947 |
ATT(2025-06,2025-03,2025-04) | -0.216117 | 0.302553 |
ATT(2025-06,2025-04,2025-05) | -0.291255 | 0.283533 |
ATT(2025-06,2025-05,2025-06) | 0.535955 | 1.060635 |
A visualization of the effects can be obtained via the plot_effects()
method.
Remark that the plot used joint confidence intervals per default.
[14]:
dml_obj.plot_effects()
/opt/hostedtoolcache/Python/3.12.10/x64/lib/python3.12/site-packages/matplotlib/cbook.py:1719: FutureWarning: Calling float on a single element Series is deprecated and will raise a TypeError in the future. Use float(ser.iloc[0]) instead
return math.isfinite(val)
[14]:
(<Figure size 1200x800 with 4 Axes>,
[<Axes: title={'center': 'First Treated: 2025-04'}, ylabel='Effect'>,
<Axes: title={'center': 'First Treated: 2025-05'}, ylabel='Effect'>,
<Axes: title={'center': 'First Treated: 2025-06'}, xlabel='Evaluation Period', ylabel='Effect'>])
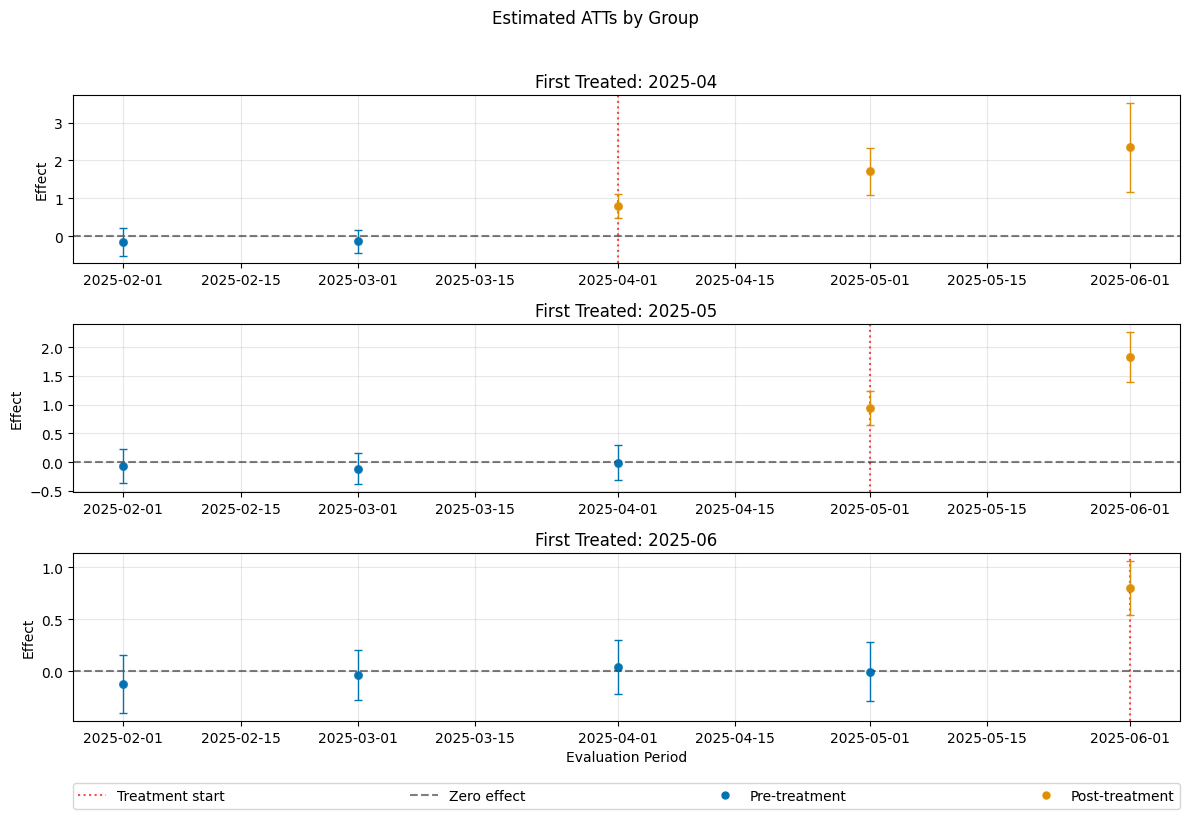
Sensitivity Analysis#
As descripted in the Sensitivity Guide, robustness checks on omitted confounding/parallel trend violations are available, via the standard sensitivity_analysis()
method.
[15]:
dml_obj.sensitivity_analysis()
print(dml_obj.sensitivity_summary)
================== Sensitivity Analysis ==================
------------------ Scenario ------------------
Significance Level: level=0.95
Sensitivity parameters: cf_y=0.03; cf_d=0.03, rho=1.0
------------------ Bounds with CI ------------------
CI lower theta lower theta theta upper \
ATT(2025-04,2025-01,2025-02) -0.461131 -0.252986 -0.149130 -0.045274
ATT(2025-04,2025-02,2025-03) -0.426144 -0.255262 -0.139825 -0.024389
ATT(2025-04,2025-03,2025-04) 0.514575 0.687499 0.795353 0.903206
ATT(2025-04,2025-03,2025-05) 1.220347 1.588211 1.715979 1.843747
ATT(2025-04,2025-03,2025-06) 1.471125 2.173390 2.344832 2.516274
ATT(2025-05,2025-01,2025-02) -0.350472 -0.181504 -0.071531 0.038441
ATT(2025-05,2025-02,2025-03) -0.381566 -0.225025 -0.112429 0.000167
ATT(2025-05,2025-03,2025-04) -0.287244 -0.118953 -0.006604 0.105745
ATT(2025-05,2025-04,2025-05) 0.668586 0.832180 0.939796 1.047411
ATT(2025-05,2025-04,2025-06) 1.417846 1.663779 1.829721 1.995664
ATT(2025-06,2025-01,2025-02) -0.390162 -0.231031 -0.126291 -0.021551
ATT(2025-06,2025-02,2025-03) -0.274782 -0.138964 -0.039415 0.060134
ATT(2025-06,2025-03,2025-04) -0.208477 -0.061564 0.043218 0.148001
ATT(2025-06,2025-04,2025-05) -0.266062 -0.104389 -0.003861 0.096666
ATT(2025-06,2025-05,2025-06) 0.540847 0.689885 0.798295 0.906705
CI upper
ATT(2025-04,2025-01,2025-02) 0.162522
ATT(2025-04,2025-02,2025-03) 0.148479
ATT(2025-04,2025-03,2025-04) 1.079930
ATT(2025-04,2025-03,2025-05) 2.186504
ATT(2025-04,2025-03,2025-06) 3.162164
ATT(2025-05,2025-01,2025-02) 0.208512
ATT(2025-05,2025-02,2025-03) 0.157689
ATT(2025-05,2025-03,2025-04) 0.276210
ATT(2025-05,2025-04,2025-05) 1.214771
ATT(2025-05,2025-04,2025-06) 2.244554
ATT(2025-06,2025-01,2025-02) 0.137646
ATT(2025-06,2025-02,2025-03) 0.197196
ATT(2025-06,2025-03,2025-04) 0.296706
ATT(2025-06,2025-04,2025-05) 0.262608
ATT(2025-06,2025-05,2025-06) 1.056718
------------------ Robustness Values ------------------
H_0 RV (%) RVa (%)
ATT(2025-04,2025-01,2025-02) 0.0 4.279334 0.000538
ATT(2025-04,2025-02,2025-03) 0.0 3.622197 0.000572
ATT(2025-04,2025-03,2025-04) 0.0 20.081050 15.798319
ATT(2025-04,2025-03,2025-05) 0.0 33.388819 22.382308
ATT(2025-04,2025-03,2025-06) 0.0 33.877211 19.956431
ATT(2025-05,2025-01,2025-02) 0.0 1.961862 0.000604
ATT(2025-05,2025-02,2025-03) 0.0 2.995688 0.000394
ATT(2025-05,2025-03,2025-04) 0.0 0.178745 0.000548
ATT(2025-05,2025-04,2025-05) 0.0 23.297008 19.553844
ATT(2025-05,2025-04,2025-06) 0.0 28.416497 24.910712
ATT(2025-06,2025-01,2025-02) 0.0 3.606018 0.000432
ATT(2025-06,2025-02,2025-03) 0.0 1.198908 0.000620
ATT(2025-06,2025-03,2025-04) 0.0 1.248617 0.000549
ATT(2025-06,2025-04,2025-05) 0.0 0.116790 0.000449
ATT(2025-06,2025-05,2025-06) 0.0 20.055206 16.568278
In this example one can clearly, distinguish the robustness of the non-zero effects vs. the pre-treatment periods.
Control Groups#
The current implementation support the following control groups
"never_treated"
"not_yet_treated"
Remark that the ``”not_yet_treated” depends on anticipation.
For differences and recommendations, we refer to Callaway and Sant’Anna(2021).
[16]:
dml_obj_nyt = DoubleMLDIDMulti(dml_data, **(default_args | {"control_group": "not_yet_treated"}))
dml_obj_nyt.fit()
dml_obj_nyt.bootstrap(n_rep_boot=5000)
dml_obj_nyt.plot_effects()
/opt/hostedtoolcache/Python/3.12.10/x64/lib/python3.12/site-packages/matplotlib/cbook.py:1719: FutureWarning: Calling float on a single element Series is deprecated and will raise a TypeError in the future. Use float(ser.iloc[0]) instead
return math.isfinite(val)
[16]:
(<Figure size 1200x800 with 4 Axes>,
[<Axes: title={'center': 'First Treated: 2025-04'}, ylabel='Effect'>,
<Axes: title={'center': 'First Treated: 2025-05'}, ylabel='Effect'>,
<Axes: title={'center': 'First Treated: 2025-06'}, xlabel='Evaluation Period', ylabel='Effect'>])
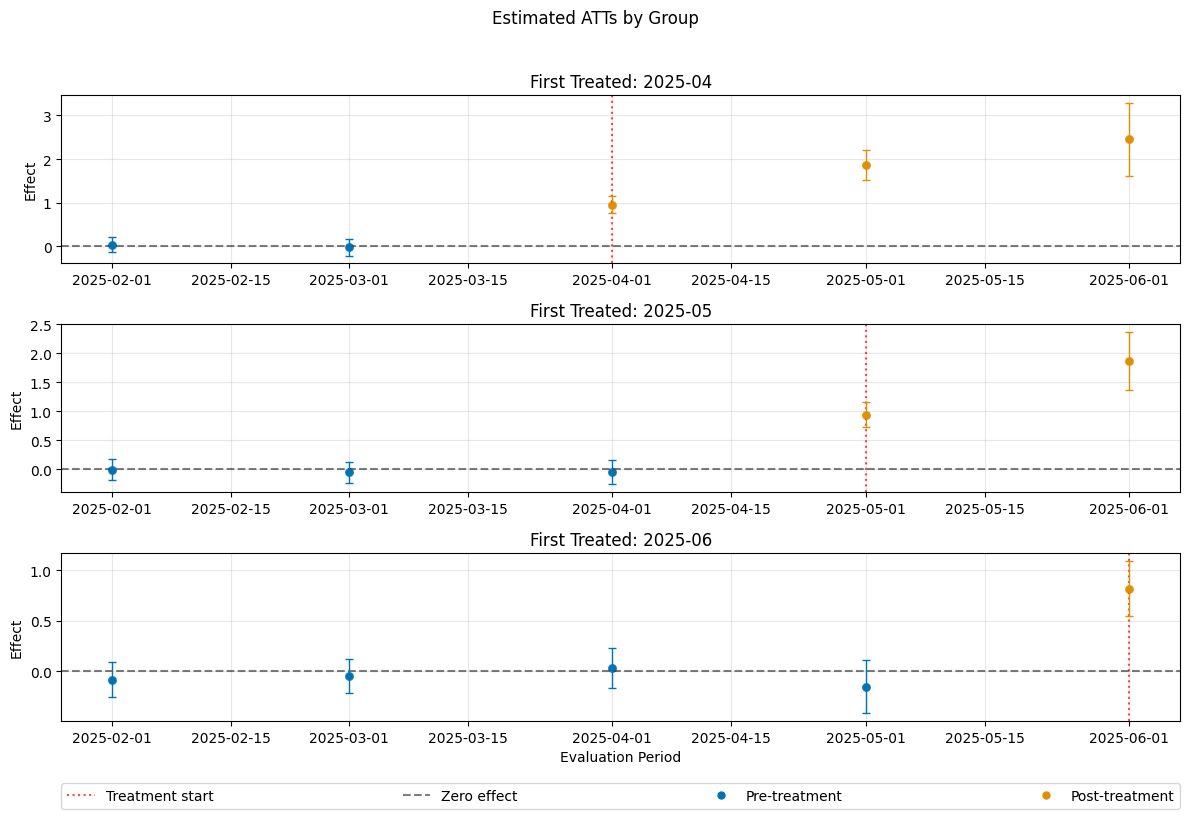
Linear Covariate Adjustment#
Remark that we relied on boosted trees to adjust for conditional parallel trends which allow for a nonlinear adjustment. In comparison to linear adjustment, we could rely on linear learners.
Remark that the DGP (``dgp_type=4``) is based on nonlinear conditional expectations such that the estimates will be biased
[17]:
linear_learners = {
"ml_g": LinearRegression(),
"ml_m": LogisticRegression(),
}
dml_obj_linear = DoubleMLDIDMulti(dml_data, **(default_args | linear_learners))
dml_obj_linear.fit()
dml_obj_linear.bootstrap(n_rep_boot=5000)
dml_obj_linear.plot_effects()
/opt/hostedtoolcache/Python/3.12.10/x64/lib/python3.12/site-packages/matplotlib/cbook.py:1719: FutureWarning: Calling float on a single element Series is deprecated and will raise a TypeError in the future. Use float(ser.iloc[0]) instead
return math.isfinite(val)
[17]:
(<Figure size 1200x800 with 4 Axes>,
[<Axes: title={'center': 'First Treated: 2025-04'}, ylabel='Effect'>,
<Axes: title={'center': 'First Treated: 2025-05'}, ylabel='Effect'>,
<Axes: title={'center': 'First Treated: 2025-06'}, xlabel='Evaluation Period', ylabel='Effect'>])
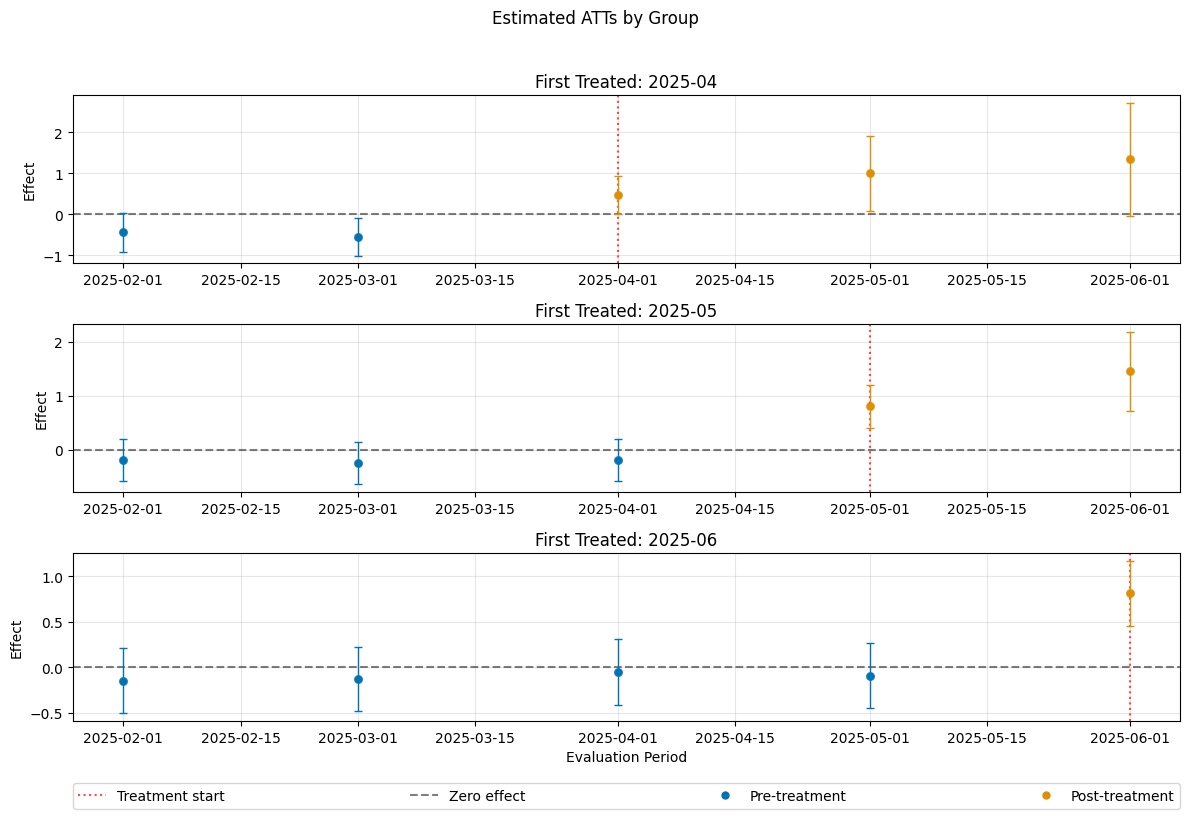
Aggregated Effects#
As the did-R-package, the \(ATT\)’s can be aggregated to summarize multiple effects. For details on different aggregations and details on their interpretations see Callaway and Sant’Anna(2021).
The aggregations are implemented via the aggregate()
method.
Group Aggregation#
To obtain group-specific effects one can would like to average \(ATT(\mathrm{g}, t_\text{eval})\) over \(t_\text{eval}\). As a sample oracle we will combine all ite
’s based on group \(\mathrm{g}\).
[18]:
df_post_treatment = df[df["t"] >= df["d"]]
df_post_treatment.groupby("d")["ite"].mean()
[18]:
d
2025-04-01 1.928383
2025-05-01 1.492782
2025-06-01 0.991117
Name: ite, dtype: float64
To obtain group-specific effects it is possible to aggregate several \(\widehat{ATT}(\mathrm{g},t_\text{pre},t_\text{eval})\) values based on the group \(\mathrm{g}\) by setting the aggregation="group"
argument.
[19]:
aggregated_group = dml_obj.aggregate(aggregation="group")
print(aggregated_group)
_ = aggregated_group.plot_effects()
================== DoubleMLDIDAggregation Object ==================
Group Aggregation
------------------ Overall Aggregated Effects ------------------
coef std err t P>|t| 2.5 % 97.5 %
1.276439 0.113732 11.22319 0.0 1.053528 1.49935
------------------ Aggregated Effects ------------------
coef std err t P>|t| 2.5 % 97.5 %
2025-04 1.618721 0.224364 7.214710 5.404566e-13 1.178976 2.058467
2025-05 1.384758 0.114610 12.082392 0.000000e+00 1.160128 1.609389
2025-06 0.798295 0.090798 8.792034 0.000000e+00 0.620335 0.976255
------------------ Additional Information ------------------
Score function: observational
Control group: never_treated
Anticipation periods: 0
/opt/hostedtoolcache/Python/3.12.10/x64/lib/python3.12/site-packages/doubleml/did/did_aggregation.py:368: UserWarning: Joint confidence intervals require bootstrapping which hasn't been performed yet. Automatically applying '.aggregated_frameworks.bootstrap(method="normal", n_rep_boot=500)' with default values. For different bootstrap settings, call bootstrap() explicitly before plotting.
warnings.warn(
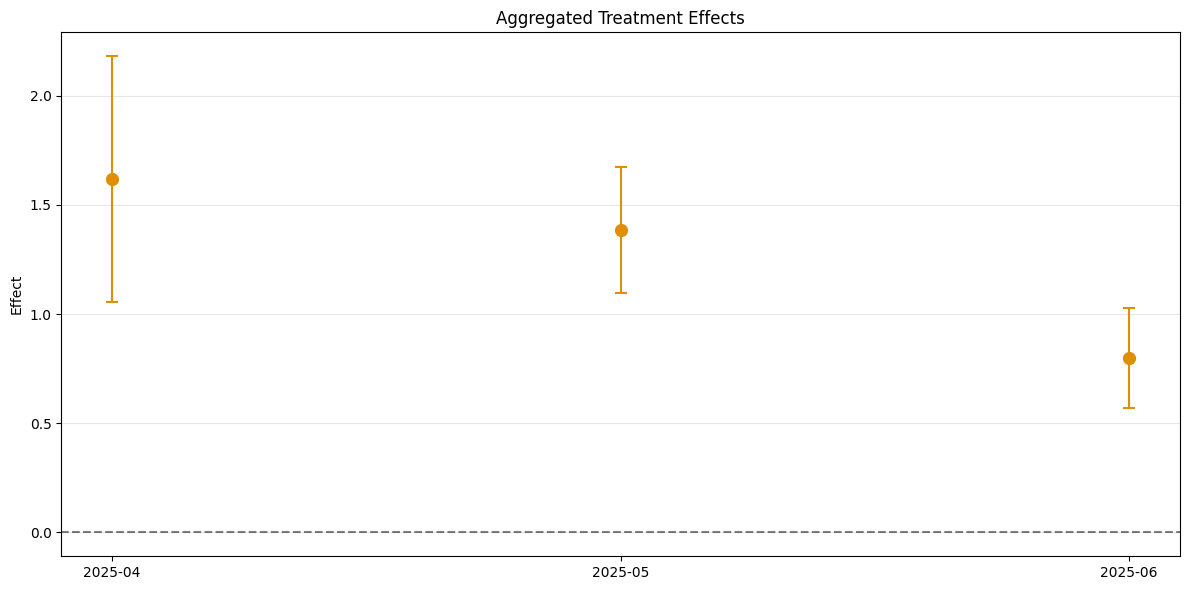
The output is a DoubleMLDIDAggregation
object which includes an overall aggregation summary based on group size.
Time Aggregation#
To obtain time-specific effects one can would like to average \(ATT(\mathrm{g}, t_\text{eval})\) over \(\mathrm{g}\) (respecting group size). As a sample oracle we will combine all ite
’s based on group \(\mathrm{g}\). As oracle values, we obtain
[20]:
df_post_treatment.groupby("t")["ite"].mean()
[20]:
t
2025-04-01 0.924024
2025-05-01 1.523703
2025-06-01 1.987602
Name: ite, dtype: float64
To aggregate \(\widehat{ATT}(\mathrm{g},t_\text{pre},t_\text{eval})\), based on \(t_\text{eval}\), but weighted with respect to group size. Corresponds to Calendar Time Effects from the did-R-package.
For calendar time effects set aggregation="time"
.
[21]:
aggregated_time = dml_obj.aggregate("time")
print(aggregated_time)
fig, ax = aggregated_time.plot_effects()
================== DoubleMLDIDAggregation Object ==================
Time Aggregation
------------------ Overall Aggregated Effects ------------------
coef std err t P>|t| 2.5 % 97.5 %
1.277412 0.126855 10.069842 0.0 1.02878 1.526044
------------------ Aggregated Effects ------------------
coef std err t P>|t| 2.5 % 97.5 %
2025-04 0.795353 0.105858 7.513365 5.773160e-14 0.587874 1.002831
2025-05 1.360044 0.138422 9.825336 0.000000e+00 1.088742 1.631347
2025-06 1.676839 0.183424 9.141876 0.000000e+00 1.317335 2.036343
------------------ Additional Information ------------------
Score function: observational
Control group: never_treated
Anticipation periods: 0
/opt/hostedtoolcache/Python/3.12.10/x64/lib/python3.12/site-packages/doubleml/did/did_aggregation.py:368: UserWarning: Joint confidence intervals require bootstrapping which hasn't been performed yet. Automatically applying '.aggregated_frameworks.bootstrap(method="normal", n_rep_boot=500)' with default values. For different bootstrap settings, call bootstrap() explicitly before plotting.
warnings.warn(
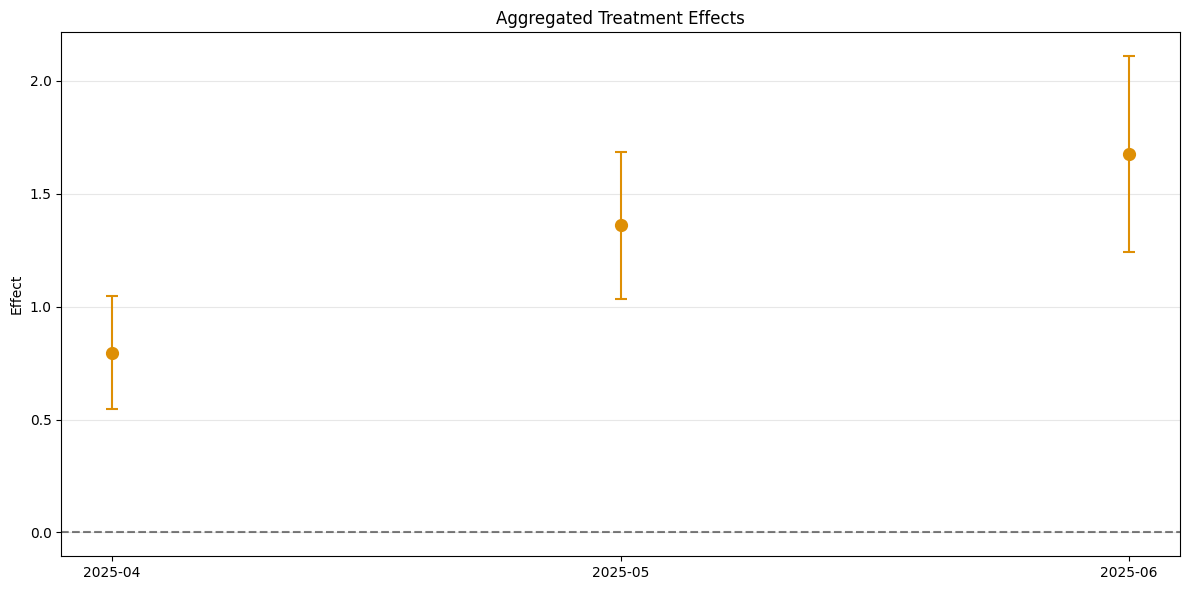
Event Study Aggregation#
To obtain event-study-type effects one can would like to aggregate \(ATT(\mathrm{g}, t_\text{eval})\) over \(e = t_\text{eval} - \mathrm{g}\) (respecting group size). As a sample oracle we will combine all ite
’s based on group \(\mathrm{g}\). As oracle values, we obtain
[22]:
df["e"] = pd.to_datetime(df["t"]).values.astype("datetime64[M]") - \
pd.to_datetime(df["d"]).values.astype("datetime64[M]")
df.groupby("e")["ite"].mean()[1:]
[22]:
e
-122 days -0.030359
-92 days 0.003671
-61 days -0.010523
-31 days -0.012147
0 days 0.967090
31 days 1.982818
59 days 2.887249
Name: ite, dtype: float64
Analogously, aggregation="eventstudy"
aggregates \(\widehat{ATT}(\mathrm{g},t_\text{pre},t_\text{eval})\) based on exposure time \(e = t_\text{eval} - \mathrm{g}\) (respecting group size).
[23]:
aggregated_eventstudy = dml_obj.aggregate("eventstudy")
print(aggregated_eventstudy)
aggregated_eventstudy.plot_effects()
================== DoubleMLDIDAggregation Object ==================
Event Study Aggregation
------------------ Overall Aggregated Effects ------------------
coef std err t P>|t| 2.5 % 97.5 %
1.651234 0.195551 8.444015 0.0 1.267962 2.034507
------------------ Aggregated Effects ------------------
coef std err t P>|t| 2.5 % 97.5 %
-4 months -0.126291 0.096670 -1.306414 1.914117e-01 -0.315761 0.063179
-3 months -0.054917 0.067547 -0.813025 4.162040e-01 -0.187306 0.077472
-2 months -0.074463 0.070502 -1.056179 2.908863e-01 -0.212645 0.063719
-1 months -0.054062 0.068191 -0.792799 4.278947e-01 -0.187715 0.079590
0 months 0.840733 0.065592 12.817609 0.000000e+00 0.712175 0.969291
1 months 1.768138 0.155289 11.386106 0.000000e+00 1.463777 2.072499
2 months 2.344832 0.406269 5.771623 7.851150e-09 1.548559 3.141105
------------------ Additional Information ------------------
Score function: observational
Control group: never_treated
Anticipation periods: 0
/opt/hostedtoolcache/Python/3.12.10/x64/lib/python3.12/site-packages/doubleml/did/did_aggregation.py:368: UserWarning: Joint confidence intervals require bootstrapping which hasn't been performed yet. Automatically applying '.aggregated_frameworks.bootstrap(method="normal", n_rep_boot=500)' with default values. For different bootstrap settings, call bootstrap() explicitly before plotting.
warnings.warn(
[23]:
(<Figure size 1200x600 with 1 Axes>,
<Axes: title={'center': 'Aggregated Treatment Effects'}, ylabel='Effect'>)
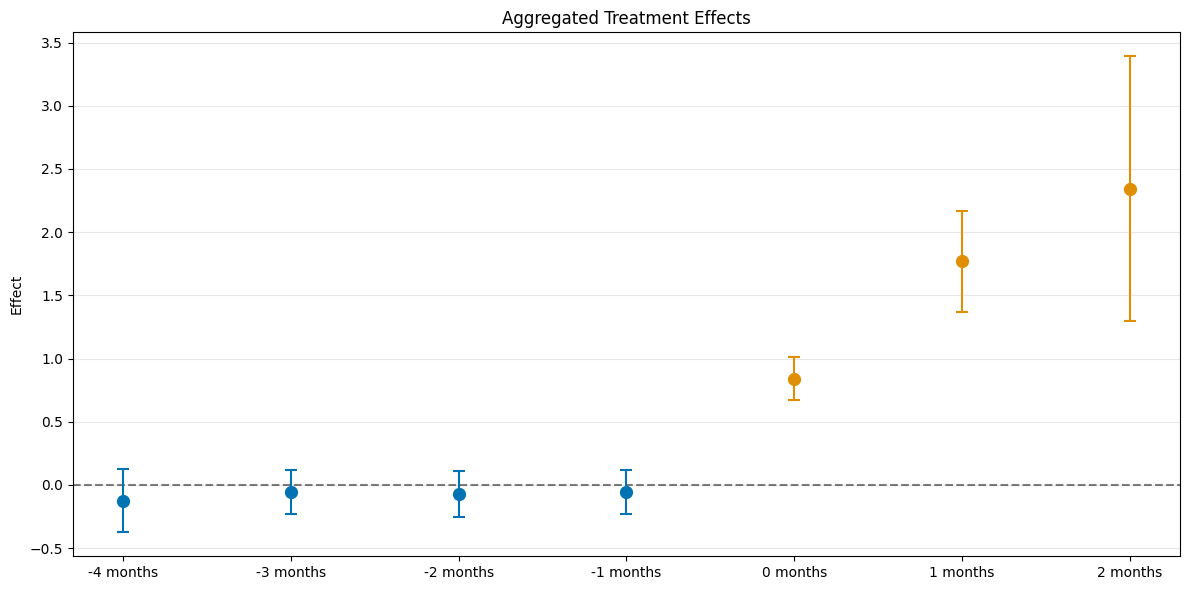
Aggregation Details#
The DoubleMLDIDAggregation
objects include several DoubleMLFrameworks
which support methods like bootstrap()
or confint()
. Further, the weights can be accessed via the properties
overall_aggregation_weights
: weights for the overall aggregationaggregation_weights
: weights for the aggregation
To clarify, e.g. for the eventstudy aggregation
[24]:
print(aggregated_eventstudy)
================== DoubleMLDIDAggregation Object ==================
Event Study Aggregation
------------------ Overall Aggregated Effects ------------------
coef std err t P>|t| 2.5 % 97.5 %
1.651234 0.195551 8.444015 0.0 1.267962 2.034507
------------------ Aggregated Effects ------------------
coef std err t P>|t| 2.5 % 97.5 %
-4 months -0.126291 0.096670 -1.306414 1.914117e-01 -0.315761 0.063179
-3 months -0.054917 0.067547 -0.813025 4.162040e-01 -0.187306 0.077472
-2 months -0.074463 0.070502 -1.056179 2.908863e-01 -0.212645 0.063719
-1 months -0.054062 0.068191 -0.792799 4.278947e-01 -0.187715 0.079590
0 months 0.840733 0.065592 12.817609 0.000000e+00 0.712175 0.969291
1 months 1.768138 0.155289 11.386106 0.000000e+00 1.463777 2.072499
2 months 2.344832 0.406269 5.771623 7.851150e-09 1.548559 3.141105
------------------ Additional Information ------------------
Score function: observational
Control group: never_treated
Anticipation periods: 0
Here, the overall effect aggregation aggregates each effect with positive exposure
[25]:
print(aggregated_eventstudy.overall_aggregation_weights)
[0. 0. 0. 0. 0.33333333 0.33333333
0.33333333]
If one would like to consider how the aggregated effect with \(e=0\) is computed, one would have to look at the corresponding set of weights within the aggregation_weights
property
[26]:
# the weights for e=0 correspond to the fifth element of the aggregation weights
aggregated_eventstudy.aggregation_weights[4]
[26]:
array([0. , 0. , 0.36301743, 0. , 0. ,
0. , 0. , 0. , 0.30746187, 0. ,
0. , 0. , 0. , 0. , 0.3295207 ])
Taking a look at the original dml_obj
, one can see that this combines the following estimates (only show month):
\(\widehat{ATT}(04,03,04)\)
\(\widehat{ATT}(05,04,05)\)
\(\widehat{ATT}(06,05,06)\)
[27]:
print(dml_obj.summary["coef"])
ATT(2025-04,2025-01,2025-02) -0.149130
ATT(2025-04,2025-02,2025-03) -0.139825
ATT(2025-04,2025-03,2025-04) 0.795353
ATT(2025-04,2025-03,2025-05) 1.715979
ATT(2025-04,2025-03,2025-06) 2.344832
ATT(2025-05,2025-01,2025-02) -0.071531
ATT(2025-05,2025-02,2025-03) -0.112429
ATT(2025-05,2025-03,2025-04) -0.006604
ATT(2025-05,2025-04,2025-05) 0.939796
ATT(2025-05,2025-04,2025-06) 1.829721
ATT(2025-06,2025-01,2025-02) -0.126291
ATT(2025-06,2025-02,2025-03) -0.039415
ATT(2025-06,2025-03,2025-04) 0.043218
ATT(2025-06,2025-04,2025-05) -0.003861
ATT(2025-06,2025-05,2025-06) 0.798295
Name: coef, dtype: float64
Anticipation#
As described in the Model Guide, one can include anticipation periods \(\delta>0\) by setting the anticipation_periods
parameter.
Data with Anticipation#
The DGP allows to include anticipation periods via the anticipation_periods
parameter. In this case the observations will be “shifted” such that units anticipate the effect earlier and the exposure effect is increased by the number of periods where the effect is anticipated.
[28]:
n_obs = 4000
n_periods = 6
df_anticipation = make_did_CS2021(n_obs, dgp_type=4, n_periods=n_periods, n_pre_treat_periods=3, time_type="datetime", anticipation_periods=1)
print(df_anticipation.shape)
df_anticipation.head()
(19038, 10)
[28]:
id | y | y0 | y1 | d | t | Z1 | Z2 | Z3 | Z4 | |
---|---|---|---|---|---|---|---|---|---|---|
1 | 0 | 226.000671 | 226.000671 | 227.785832 | 2025-05-01 | 2025-01-01 | 0.56546 | 0.498331 | 1.321175 | 0.915738 |
2 | 0 | 239.594137 | 239.594137 | 238.709283 | 2025-05-01 | 2025-02-01 | 0.56546 | 0.498331 | 1.321175 | 0.915738 |
3 | 0 | 248.036755 | 248.036755 | 247.092654 | 2025-05-01 | 2025-03-01 | 0.56546 | 0.498331 | 1.321175 | 0.915738 |
4 | 0 | 260.234067 | 258.626122 | 260.234067 | 2025-05-01 | 2025-04-01 | 0.56546 | 0.498331 | 1.321175 | 0.915738 |
5 | 0 | 270.146933 | 268.007132 | 270.146933 | 2025-05-01 | 2025-05-01 | 0.56546 | 0.498331 | 1.321175 | 0.915738 |
To visualize the anticipation, we will again plot the “oracle” values
[29]:
df_anticipation["ite"] = df_anticipation["y1"] - df_anticipation["y0"]
df_anticipation["First Treated"] = df_anticipation["d"].dt.strftime("%Y-%m").fillna("Never Treated")
agg_df_anticipation = df_anticipation.groupby(["t", "First Treated"]).agg(**agg_dictionary).reset_index()
agg_df_anticipation.head()
[29]:
t | First Treated | y_mean | y_lower_quantile | y_upper_quantile | ite_mean | ite_lower_quantile | ite_upper_quantile | |
---|---|---|---|---|---|---|---|---|
0 | 2025-01-01 | 2025-04 | 209.003285 | 192.937904 | 225.148513 | -0.042595 | -2.327865 | 2.298648 |
1 | 2025-01-01 | 2025-05 | 210.487010 | 194.459493 | 227.912536 | -0.087792 | -2.428659 | 2.120952 |
2 | 2025-01-01 | 2025-06 | 213.429960 | 196.541694 | 230.447361 | 0.039747 | -2.344718 | 2.241619 |
3 | 2025-01-01 | Never Treated | 217.818353 | 200.646634 | 234.434920 | 0.050192 | -2.299754 | 2.483817 |
4 | 2025-02-01 | 2025-04 | 209.033142 | 185.544049 | 233.633004 | -0.073080 | -2.409718 | 2.224007 |
One can see that the effect is already anticipated one period before the actual treatment assignment.
[30]:
plot_data(agg_df_anticipation, col_name='ite')
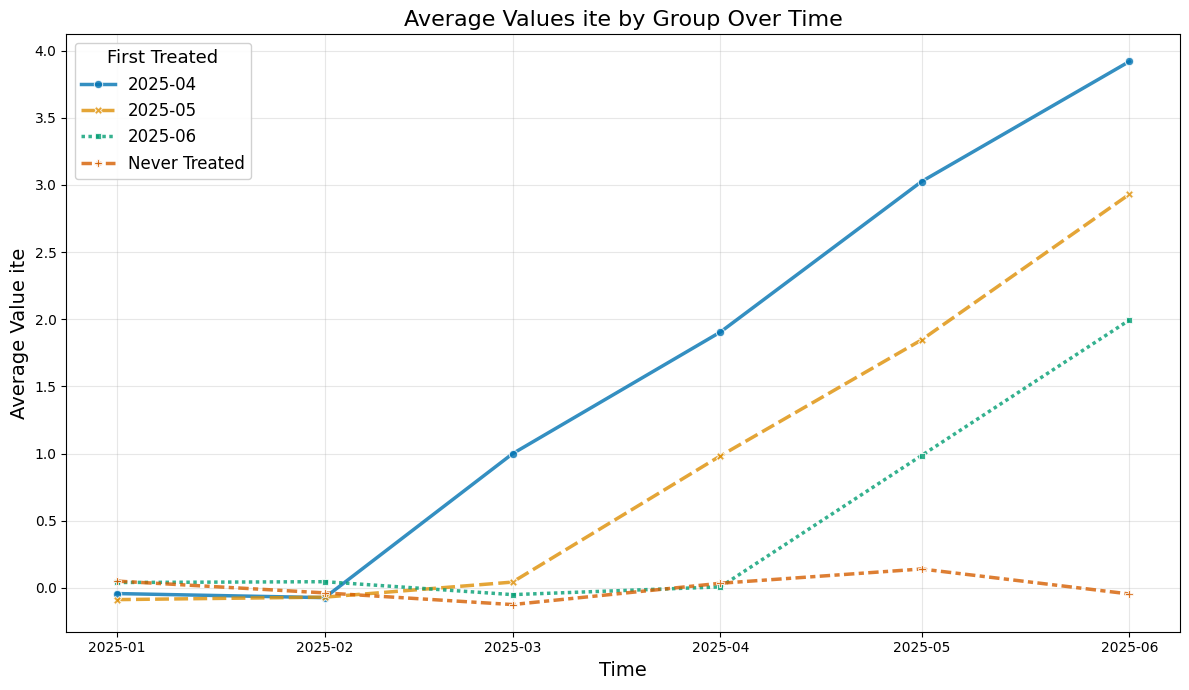
Initialize a corresponding DoubleMLPanelData
object.
[31]:
dml_data_anticipation = DoubleMLPanelData(
data=df_anticipation,
y_col="y",
d_cols="d",
id_col="id",
t_col="t",
x_cols=["Z1", "Z2", "Z3", "Z4"],
datetime_unit="M"
)
ATT Estimation#
Let us take a look at the estimation without anticipation.
[32]:
dml_obj_anticipation = DoubleMLDIDMulti(dml_data_anticipation, **default_args)
dml_obj_anticipation.fit()
dml_obj_anticipation.bootstrap(n_rep_boot=5000)
dml_obj_anticipation.plot_effects()
/opt/hostedtoolcache/Python/3.12.10/x64/lib/python3.12/site-packages/doubleml/double_ml.py:1470: UserWarning: The estimated nu2 for d is not positive. Re-estimation based on riesz representer (non-orthogonal).
warnings.warn(msg, UserWarning)
/opt/hostedtoolcache/Python/3.12.10/x64/lib/python3.12/site-packages/matplotlib/cbook.py:1719: FutureWarning: Calling float on a single element Series is deprecated and will raise a TypeError in the future. Use float(ser.iloc[0]) instead
return math.isfinite(val)
[32]:
(<Figure size 1200x800 with 4 Axes>,
[<Axes: title={'center': 'First Treated: 2025-04'}, ylabel='Effect'>,
<Axes: title={'center': 'First Treated: 2025-05'}, ylabel='Effect'>,
<Axes: title={'center': 'First Treated: 2025-06'}, xlabel='Evaluation Period', ylabel='Effect'>])
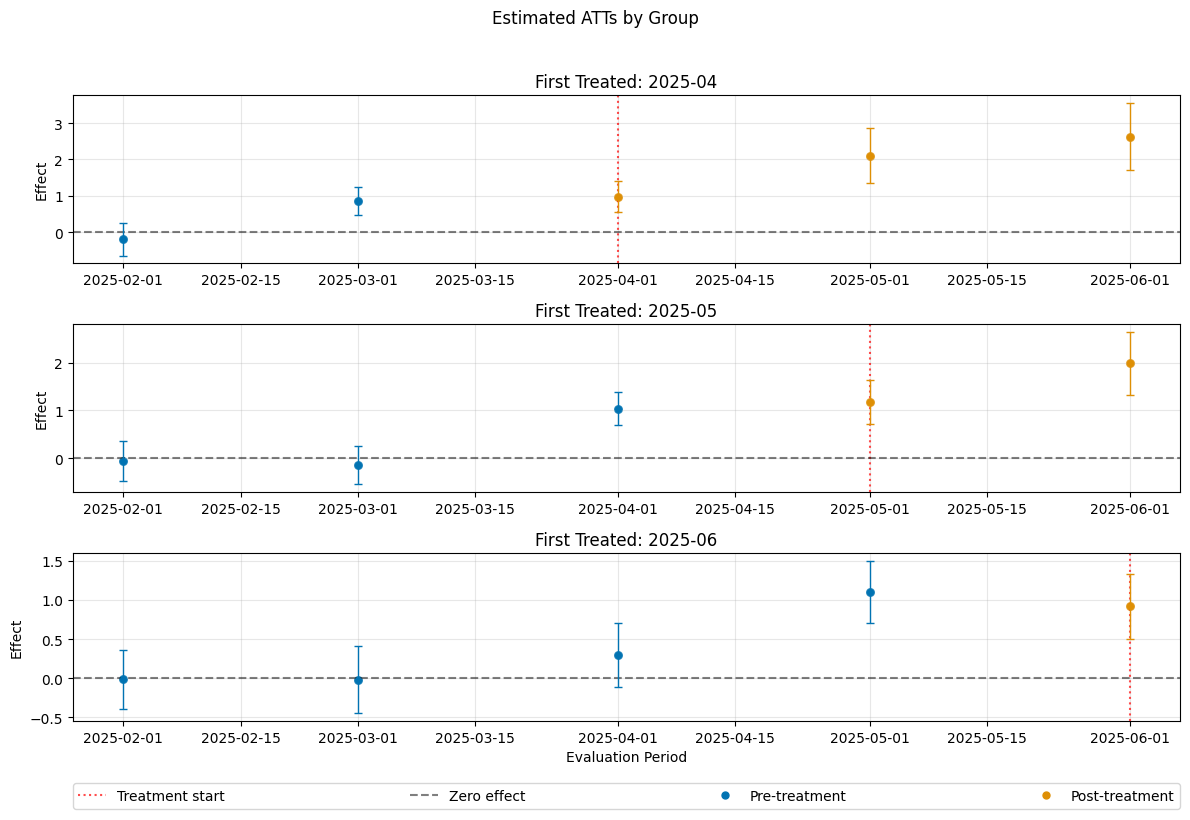
The effects are obviously biased. To include anticipation periods, one can adjust the anticipation_periods
parameter. Correspondingly, the outcome regression (and not yet treated units) are adjusted.
[33]:
dml_obj_anticipation = DoubleMLDIDMulti(dml_data_anticipation, **(default_args| {"anticipation_periods": 1}))
dml_obj_anticipation.fit()
dml_obj_anticipation.bootstrap(n_rep_boot=5000)
dml_obj_anticipation.plot_effects()
/opt/hostedtoolcache/Python/3.12.10/x64/lib/python3.12/site-packages/doubleml/double_ml.py:1470: UserWarning: The estimated nu2 for d is not positive. Re-estimation based on riesz representer (non-orthogonal).
warnings.warn(msg, UserWarning)
/opt/hostedtoolcache/Python/3.12.10/x64/lib/python3.12/site-packages/matplotlib/cbook.py:1719: FutureWarning: Calling float on a single element Series is deprecated and will raise a TypeError in the future. Use float(ser.iloc[0]) instead
return math.isfinite(val)
/opt/hostedtoolcache/Python/3.12.10/x64/lib/python3.12/site-packages/matplotlib/cbook.py:1719: FutureWarning: Calling float on a single element Series is deprecated and will raise a TypeError in the future. Use float(ser.iloc[0]) instead
return math.isfinite(val)
[33]:
(<Figure size 1200x800 with 4 Axes>,
[<Axes: title={'center': 'First Treated: 2025-04'}, ylabel='Effect'>,
<Axes: title={'center': 'First Treated: 2025-05'}, ylabel='Effect'>,
<Axes: title={'center': 'First Treated: 2025-06'}, xlabel='Evaluation Period', ylabel='Effect'>])
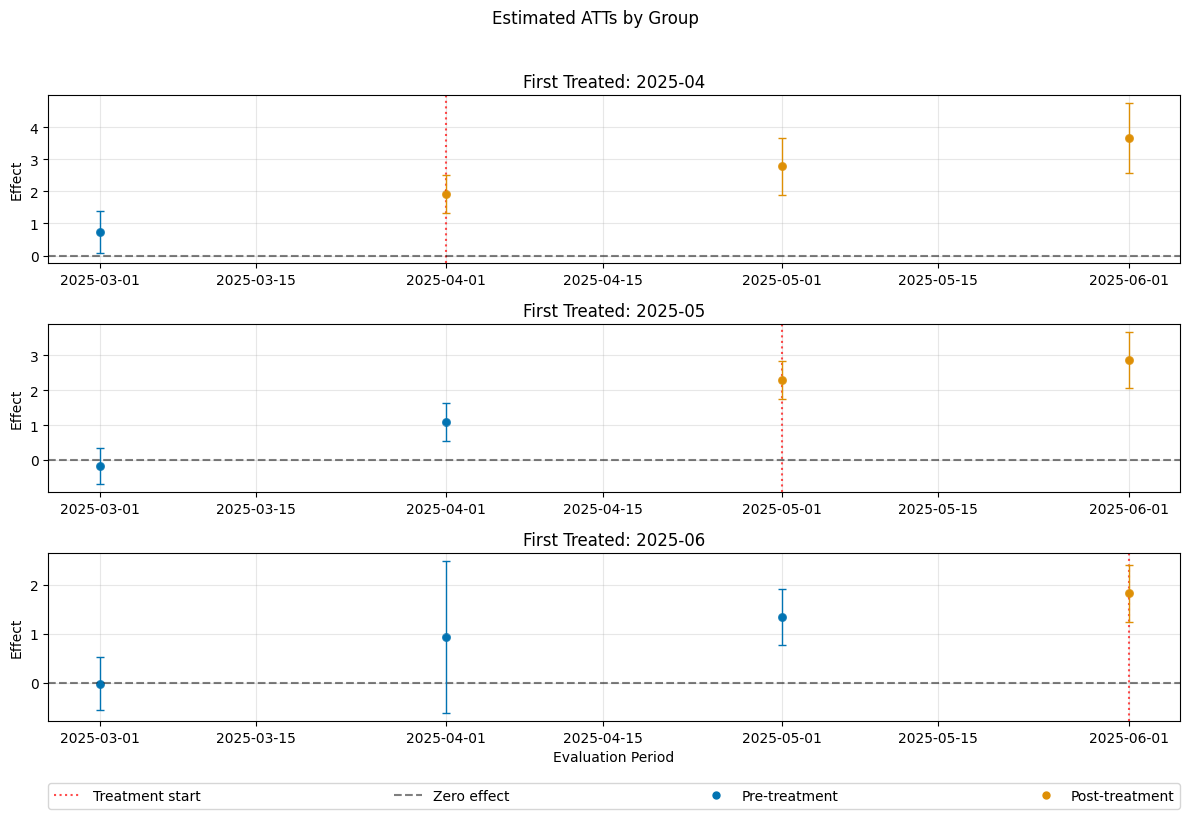
Group-Time Combinations#
The default option gt_combinations="standard"
includes all group time values with the specific choice of \(t_\text{pre} = \min(\mathrm{g}, t_\text{eval}) - 1\) (without anticipation) which is the weakest possible parallel trend assumption.
Other options are possible or only specific combinations of \((\mathrm{g},t_\text{pre},t_\text{eval})\).
All combinations#
The option gt_combinations="all"
includes all relevant group time values with \(t_\text{pre} < \min(\mathrm{g}, t_\text{eval})\), including longer parallel trend assumptions. This can result in multiple estimates for the same \(ATT(\mathrm{g},t)\), which have slightly different assumptions (length of parallel trends).
[34]:
dml_obj_all = DoubleMLDIDMulti(dml_data, **(default_args| {"gt_combinations": "all"}))
dml_obj_all.fit()
dml_obj_all.bootstrap(n_rep_boot=5000)
dml_obj_all.plot_effects()
[34]:
(<Figure size 1200x800 with 4 Axes>,
[<Axes: title={'center': 'First Treated: 2025-04'}, ylabel='Effect'>,
<Axes: title={'center': 'First Treated: 2025-05'}, ylabel='Effect'>,
<Axes: title={'center': 'First Treated: 2025-06'}, xlabel='Evaluation Period', ylabel='Effect'>])
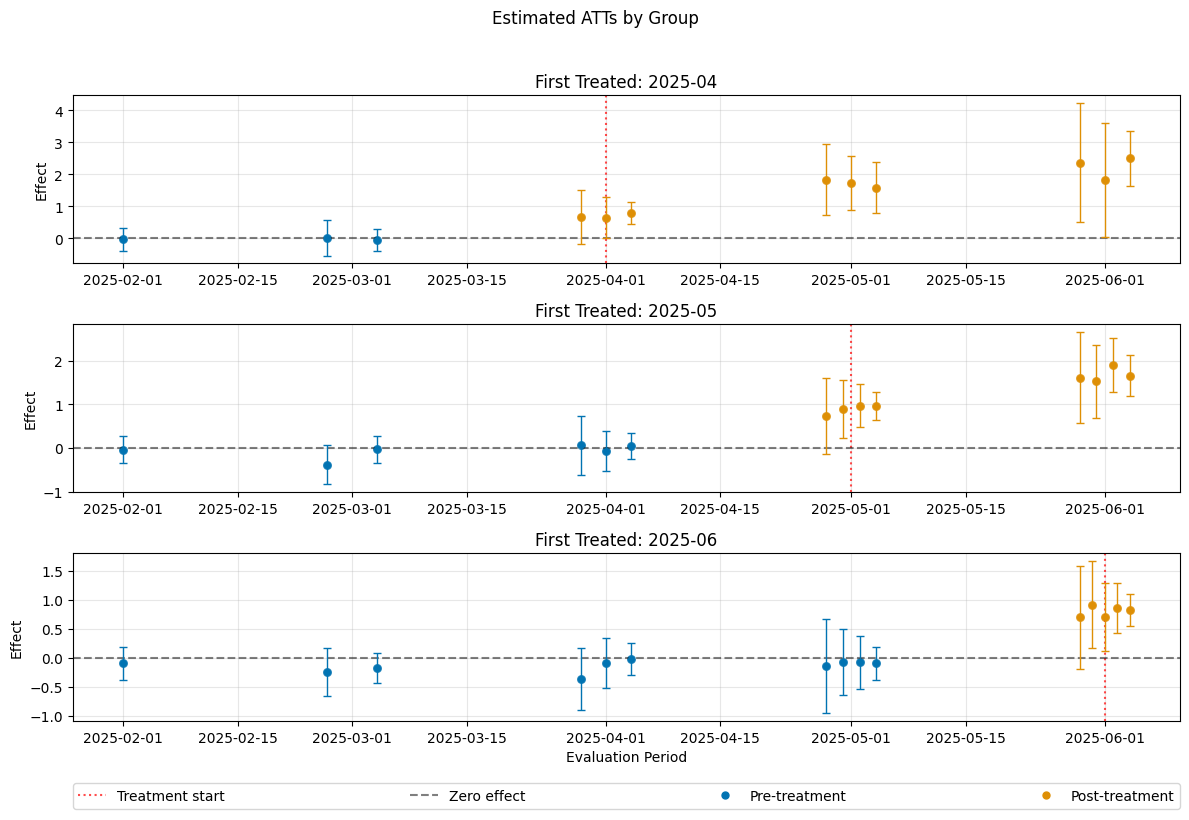
Selected Combinations#
Instead it is also possible to just submit a list of tuples containing \((\mathrm{g}, t_\text{pre}, t_\text{eval})\) combinations. E.g. only two combinations
[35]:
gt_dict = {
"gt_combinations": [
(np.datetime64('2025-04'),
np.datetime64('2025-01'),
np.datetime64('2025-02')),
(np.datetime64('2025-04'),
np.datetime64('2025-02'),
np.datetime64('2025-03')),
]
}
dml_obj_all = DoubleMLDIDMulti(dml_data, **(default_args| gt_dict))
dml_obj_all.fit()
dml_obj_all.bootstrap(n_rep_boot=5000)
dml_obj_all.plot_effects()
[35]:
(<Figure size 1200x800 with 2 Axes>,
[<Axes: title={'center': 'First Treated: 2025-04'}, xlabel='Evaluation Period', ylabel='Effect'>])
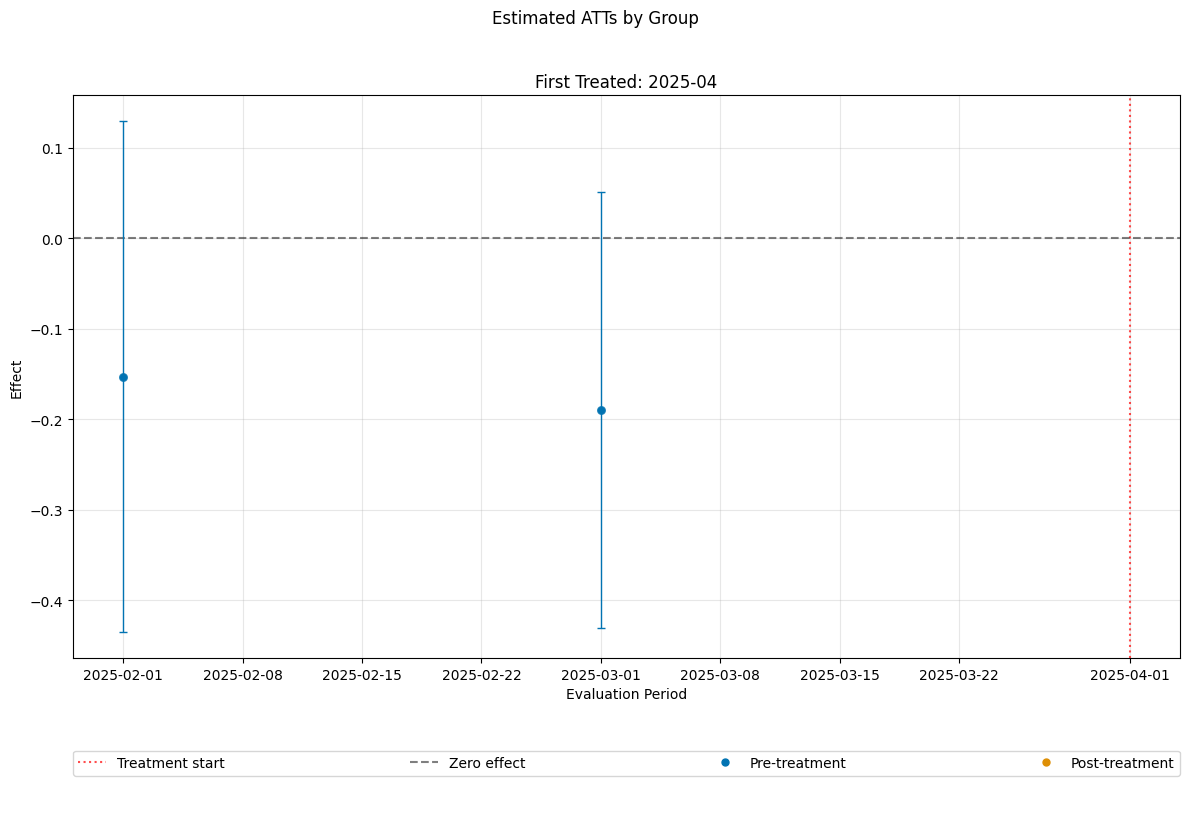