Note
-
Download Jupyter notebook:
https://docs.doubleml.org/stable/examples/py_double_ml_pension_qte.ipynb.
Python: Impact of 401(k) on Financial Wealth (Quantile Effects)#
In this real-data example, we illustrate how the DoubleML package can be used to estimate the effect of 401(k) eligibility and participation on accumulated assets. The 401(k) data set has been analyzed in several studies, among others Chernozhukov et al. (2018), see Kallus et al. (2019) for quantile effects.
Remark: This notebook focuses on the evaluation of the treatment effect at different quantiles. For a basic introduction to the DoubleML package and a detailed example of the average treatment effect estimation for the 401(k) data set, we refer to the notebook Python: Impact of 401(k) on Financial Wealth. The Data sections of both notebooks coincide.
401(k) plans are pension accounts sponsored by employers. The key problem in determining the effect of participation in 401(k) plans on accumulated assets is saver heterogeneity coupled with the fact that the decision to enroll in a 401(k) is non-random. It is generally recognized that some people have a higher preference for saving than others. It also seems likely that those individuals with high unobserved preference for saving would be most likely to choose to participate in tax-advantaged retirement savings plans and would tend to have otherwise high amounts of accumulated assets. The presence of unobserved savings preferences with these properties then implies that conventional estimates that do not account for saver heterogeneity and endogeneity of participation will be biased upward, tending to overstate the savings effects of 401(k) participation.
One can argue that eligibility for enrolling in a 401(k) plan in this data can be taken as exogenous after conditioning on a few observables of which the most important for their argument is income. The basic idea is that, at least around the time 401(k)’s initially became available, people were unlikely to be basing their employment decisions on whether an employer offered a 401(k) but would instead focus on income and other aspects of the job.
Data#
The preprocessed data can be fetched by calling fetch_401K(). Note that an internet connection is required for loading the data.
[1]:
import numpy as np
import pandas as pd
import doubleml as dml
import multiprocessing
from doubleml.datasets import fetch_401K
from sklearn.base import clone
from lightgbm import LGBMClassifier, LGBMRegressor
import matplotlib.pyplot as plt
import seaborn as sns
[2]:
sns.set()
colors = sns.color_palette()
[3]:
plt.rcParams['figure.figsize'] = 10., 7.5
sns.set(font_scale=1.5)
sns.set_style('whitegrid', {'axes.spines.top': False,
'axes.spines.bottom': False,
'axes.spines.left': False,
'axes.spines.right': False})
[4]:
data = fetch_401K(return_type='DataFrame')
[5]:
print(data.describe())
nifa net_tfa tw age inc \
count 9.915000e+03 9.915000e+03 9.915000e+03 9915.000000 9915.000000
mean 1.392864e+04 1.805153e+04 6.381685e+04 41.060212 37200.621094
std 5.490504e+04 6.352259e+04 1.115296e+05 10.344505 24774.251953
min 0.000000e+00 -5.023020e+05 -5.023020e+05 25.000000 -2652.000000
25% 2.000000e+02 -5.000000e+02 3.291500e+03 32.000000 19413.000000
50% 1.635000e+03 1.499000e+03 2.510000e+04 40.000000 31476.000000
75% 8.765500e+03 1.652450e+04 8.148750e+04 48.000000 48583.500000
max 1.430298e+06 1.536798e+06 2.029910e+06 64.000000 242124.000000
fsize educ db marr twoearn \
count 9915.000000 9915.000000 9915.000000 9915.000000 9915.000000
mean 2.865860 13.206253 0.271004 0.604841 0.380837
std 1.538937 2.810382 0.444500 0.488909 0.485617
min 1.000000 1.000000 0.000000 0.000000 0.000000
25% 2.000000 12.000000 0.000000 0.000000 0.000000
50% 3.000000 12.000000 0.000000 1.000000 0.000000
75% 4.000000 16.000000 1.000000 1.000000 1.000000
max 13.000000 18.000000 1.000000 1.000000 1.000000
e401 p401 pira hown
count 9915.000000 9915.000000 9915.000000 9915.000000
mean 0.371357 0.261624 0.242158 0.635199
std 0.483192 0.439541 0.428411 0.481399
min 0.000000 0.000000 0.000000 0.000000
25% 0.000000 0.000000 0.000000 0.000000
50% 0.000000 0.000000 0.000000 1.000000
75% 1.000000 1.000000 0.000000 1.000000
max 1.000000 1.000000 1.000000 1.000000
The data consist of 9,915 observations at the household level drawn from the 1991 Survey of Income and Program Participation (SIPP). All the variables are referred to 1990. We use net financial assets (net_tfa) as the outcome variable, \(Y\), in our analysis. The net financial assets are computed as the sum of IRA balances, 401(k) balances, checking accounts, saving bonds, other interest-earning accounts, other interest-earning assets, stocks, and mutual funds less non mortgage debts.
Among the \(9915\) individuals, \(3682\) are eligible to participate in the program. The variable e401 indicates eligibility and p401 indicates participation, respectively.
At first consider eligibility as the treatment and define the following data.
[6]:
# Set up basic model: Specify variables for data-backend
features_base = ['age', 'inc', 'educ', 'fsize', 'marr',
'twoearn', 'db', 'pira', 'hown']
# Initialize DoubleMLData (data-backend of DoubleML)
data_dml_base = dml.DoubleMLData(data,
y_col='net_tfa',
d_cols='e401',
x_cols=features_base)
Estimating Potential Quantiles and Quantile Treatment Effects#
We will use the DoubleML package to estimate quantile treatment effects of 401(k) eligibility, i.e. e401
. As it is more interesting to take a look at a range of quantiles instead of a single one, we will first define a discretisized grid of quanitles tau_vec
, which will range from the 10%-quantile to the 90%-quantile. Further, we need a machine learning algorithm to estimate the nuisance elements of our model. In this example, we will use
a basic LGBMClassifier
.
[7]:
tau_vec = np.arange(0.1,0.95,0.05)
n_folds = 5
# Learners
class_learner = LGBMClassifier(n_estimators=300, learning_rate=0.05, num_leaves=10)
reg_learner = LGBMRegressor(n_estimators=300, learning_rate=0.05, num_leaves=10)
Next, we will apply create an DoubleMLPQ
object for each quantile to fit a quantile model. Here, we have to specifiy, whether we would like to estimate a potential quantile for the treatment group treatment=1
or control treatment=0
. Further basic options are trimming and normalization of the propensity scores (trimming_rule="truncate"
, trimming_threshold=0.01
and normalize_ipw=True
).
[8]:
PQ_0 = np.full((len(tau_vec)), np.nan)
PQ_1 = np.full((len(tau_vec)), np.nan)
ci_PQ_0 = np.full((len(tau_vec),2), np.nan)
ci_PQ_1 = np.full((len(tau_vec),2), np.nan)
for idx_tau, tau in enumerate(tau_vec):
print(f'Quantile: {tau}')
dml_PQ_0 = dml.DoubleMLPQ(data_dml_base,
ml_g=clone(class_learner),
ml_m=clone(class_learner),
score="PQ",
treatment=0,
quantile=tau,
n_folds=n_folds,
normalize_ipw=True,
trimming_rule="truncate",
trimming_threshold=1e-2)
dml_PQ_1 = dml.DoubleMLPQ(data_dml_base,
ml_g=clone(class_learner),
ml_m=clone(class_learner),
score="PQ",
treatment=1,
quantile=tau,
n_folds=n_folds,
normalize_ipw=True,
trimming_rule="truncate",
trimming_threshold=1e-2)
dml_PQ_0.fit()
dml_PQ_1.fit()
PQ_0[idx_tau] = dml_PQ_0.coef
PQ_1[idx_tau] = dml_PQ_1.coef
ci_PQ_0[idx_tau, :] = dml_PQ_0.confint(level=0.95).to_numpy()
ci_PQ_1[idx_tau, :] = dml_PQ_1.confint(level=0.95).to_numpy()
Quantile: 0.1
Quantile: 0.15000000000000002
Quantile: 0.20000000000000004
Quantile: 0.25000000000000006
Quantile: 0.30000000000000004
Quantile: 0.3500000000000001
Quantile: 0.40000000000000013
Quantile: 0.45000000000000007
Quantile: 0.5000000000000001
Quantile: 0.5500000000000002
Quantile: 0.6000000000000002
Quantile: 0.6500000000000001
Quantile: 0.7000000000000002
Quantile: 0.7500000000000002
Quantile: 0.8000000000000002
Quantile: 0.8500000000000002
Quantile: 0.9000000000000002
Additionally, each DoubleMLPQ
object has a (hopefully) helpful summary, which indicates also the evaluation of the nuisance elements with cross-validated estimation. See e.g. `dml_PQ_1’
[9]:
print(dml_PQ_1)
================== DoubleMLPQ Object ==================
------------------ Data summary ------------------
Outcome variable: net_tfa
Treatment variable(s): ['e401']
Covariates: ['age', 'inc', 'educ', 'fsize', 'marr', 'twoearn', 'db', 'pira', 'hown']
Instrument variable(s): None
No. Observations: 9915
------------------ Score & algorithm ------------------
Score function: PQ
DML algorithm: dml2
------------------ Machine learner ------------------
Learner ml_g: LGBMClassifier(learning_rate=0.05, n_estimators=300, num_leaves=10)
Learner ml_m: LGBMClassifier(learning_rate=0.05, n_estimators=300, num_leaves=10)
Out-of-sample Performance:
Learner ml_g RMSE: [[0.31337878]]
Learner ml_m RMSE: [[0.4449272]]
------------------ Resampling ------------------
No. folds: 5
No. repeated sample splits: 1
Apply cross-fitting: True
------------------ Fit summary ------------------
coef std err t P>|t| 2.5 % \
e401 63499.0 1855.668337 34.218938 1.264274e-256 59861.956892
97.5 %
e401 67136.043108
Finally, let us take a look at the estimated potential quantiles
[10]:
data_pq = {"Quantile": tau_vec,
"DML Y(0)": PQ_0, "DML Y(1)": PQ_1,
"DML Y(0) lower": ci_PQ_0[:, 0], "DML Y(0) upper": ci_PQ_0[:, 1],
"DML Y(1) lower": ci_PQ_1[:, 0], "DML Y(1) upper": ci_PQ_1[:, 1]}
df_pq = pd.DataFrame(data_pq)
print(df_pq)
Quantile DML Y(0) DML Y(1) DML Y(0) lower DML Y(0) upper \
0 0.10 -5.150000e+03 -4200.0 -5518.552508 -4781.447492
1 0.15 -3.197000e+03 -2000.0 -3420.763691 -2973.236309
2 0.20 -1.900000e+03 -733.0 -2061.872222 -1738.127778
3 0.25 -9.910000e+02 -6.0 -1129.758391 -852.241609
4 0.30 -3.310000e+02 201.0 -467.454081 -194.545919
5 0.35 -9.880808e-13 1000.0 -140.411447 140.411447
6 0.40 8.262423e-13 1586.0 -141.518446 141.518446
7 0.45 1.490000e+02 2927.0 6.781233 291.218767
8 0.50 5.000000e+02 5250.0 354.965774 645.034226
9 0.55 1.200000e+03 6530.0 1038.506687 1361.493313
10 0.60 2.318000e+03 10000.0 2103.647002 2532.352998
11 0.65 4.100000e+03 13300.0 3710.041459 4489.958541
12 0.70 6.750000e+03 18500.0 6029.711024 7470.288976
13 0.75 1.052000e+04 24199.0 9551.134146 11488.865854
14 0.80 1.650000e+04 33500.0 14984.664147 18015.335853
15 0.85 2.600000e+04 45500.0 23748.752283 28251.247717
16 0.90 4.144500e+04 63499.0 37939.488460 44950.511540
DML Y(1) lower DML Y(1) upper
0 -4835.847966 -3564.152034
1 -2439.318552 -1560.681448
2 -1238.098317 -227.901683
3 -472.478032 460.478032
4 -343.083750 745.083750
5 552.392400 1447.607600
6 1107.286593 2064.713407
7 2036.542333 3817.457667
8 4389.402902 6110.597098
9 5702.335176 7357.664824
10 8440.555150 11559.444850
11 11932.311253 14667.688747
12 16725.272296 20274.727704
13 22222.986383 26175.013617
14 30383.148802 36616.851198
15 42338.762748 48661.237252
16 59861.956892 67136.043108
[11]:
from matplotlib import pyplot as plt
plt.rcParams['figure.figsize'] = 10., 7.5
fig, (ax1, ax2) = plt.subplots(1 ,2)
ax1.grid(visible=True); ax2.grid(visible=True)
ax1.plot(df_pq['Quantile'],df_pq['DML Y(0)'], color='violet', label='Estimated Quantile Y(0)')
ax1.fill_between(df_pq['Quantile'], df_pq['DML Y(0) lower'], df_pq['DML Y(0) upper'], color='violet', alpha=.3, label='Confidence Interval')
ax1.legend()
ax2.plot(df_pq['Quantile'],df_pq['DML Y(1)'], color='violet', label='Estimated Quantile Y(1)')
ax2.fill_between(df_pq['Quantile'], df_pq['DML Y(1) lower'], df_pq['DML Y(1) upper'], color='violet', alpha=.3, label='Confidence Interval')
ax2.legend()
fig.suptitle('Potential Quantiles', fontsize=16)
fig.supxlabel('Quantile')
_ = fig.supylabel('Potential Quantile and 95%-CI')
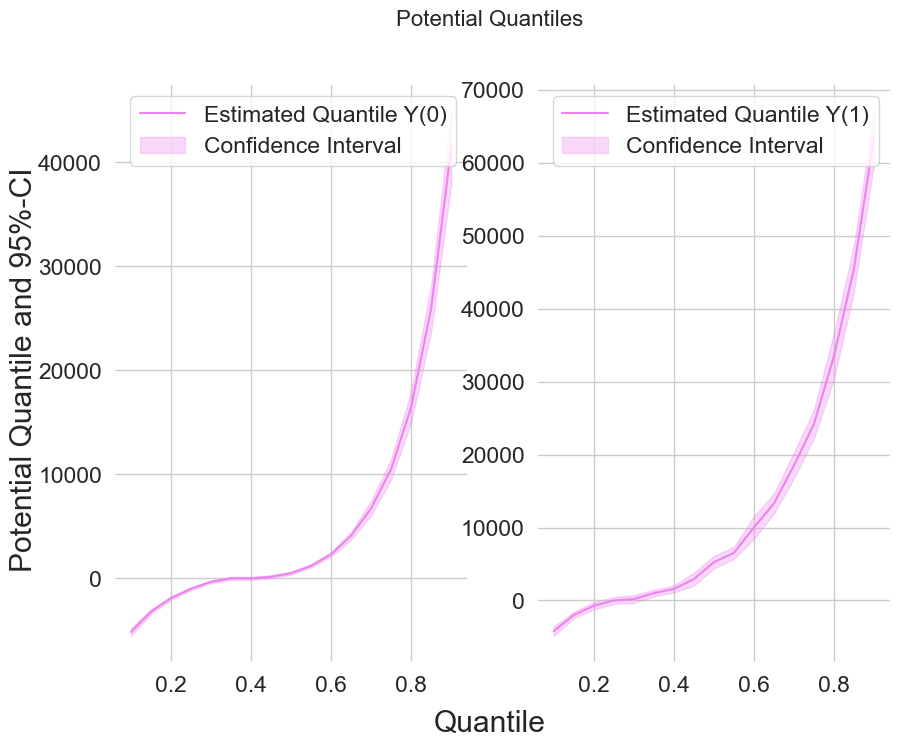
As we are interested in the QTE, we can use the DoubleMLQTE
object, which internally fits two DoubleMLPQ
objects for the treatment and control group. The main advantage is to apply this to a list of quantiles and construct uniformly valid confidence intervals for the range of treatment effects.
[12]:
n_cores = multiprocessing.cpu_count()
cores_used = np.min([5, n_cores - 1])
print(f"Number of Cores used: {cores_used}")
np.random.seed(42)
dml_QTE = dml.DoubleMLQTE(data_dml_base,
ml_g=clone(class_learner),
ml_m=clone(class_learner),
quantiles=tau_vec,
score='PQ',
n_folds=n_folds,
normalize_ipw=True,
trimming_rule="truncate",
trimming_threshold=1e-2)
dml_QTE.fit(n_jobs_models=cores_used)
print(dml_QTE)
Number of Cores used: 5
================== DoubleMLQTE Object ==================
------------------ Fit summary ------------------
coef std err t P>|t| 2.5 % \
0.10 1210.0 486.438569 2.487467 1.286563e-02 256.597923
0.15 1230.0 263.748513 4.663533 3.108257e-06 713.062414
0.20 1211.0 251.948868 4.806531 1.535718e-06 717.189293
0.25 1000.0 244.841847 4.084269 4.421576e-05 520.118799
0.30 622.0 255.252133 2.436806 1.481761e-02 121.715013
0.35 1031.0 274.813682 3.751633 1.756867e-04 492.375081
0.40 2006.0 320.163566 6.265547 3.715180e-10 1378.490941
0.45 3329.0 427.336461 7.790115 6.694845e-15 2491.435927
0.50 4601.0 448.109454 10.267581 9.864741e-25 3722.721609
0.55 6000.0 588.816752 10.189927 2.199282e-24 4845.940373
0.60 7040.0 605.739720 11.622153 3.180176e-31 5852.771965
0.65 9223.0 804.541821 11.463668 2.008266e-30 7646.127006
0.70 10928.0 859.705581 12.711328 5.115792e-37 9243.008023
0.75 12410.0 1018.114834 12.189195 3.549109e-34 10414.531594
0.80 16590.0 1589.396531 10.437924 1.664103e-25 13474.840041
0.85 19382.0 1622.701413 11.944280 6.955005e-33 16201.563673
0.90 21550.0 2279.055439 9.455672 3.209546e-21 17083.133421
97.5 %
0.10 2163.402077
0.15 1746.937586
0.20 1704.810707
0.25 1479.881201
0.30 1122.284987
0.35 1569.624919
0.40 2633.509059
0.45 4166.564073
0.50 5479.278391
0.55 7154.059627
0.60 8227.228035
0.65 10799.872994
0.70 12612.991977
0.75 14405.468406
0.80 19705.159959
0.85 22562.436327
0.90 26016.866579
For uniformly valid confidence intervals, we still need to apply a bootstrap first. Let’s take a quick look at the QTEs combinded with a confidence interval.
[13]:
dml_QTE.bootstrap(n_rep_boot=2000)
ci_QTE = dml_QTE.confint(level=0.95, joint=True)
data_qte = {"Quantile": tau_vec, "DML QTE": dml_QTE.coef,
"DML QTE lower": ci_QTE["2.5 %"], "DML QTE upper": ci_QTE["97.5 %"]}
df_qte = pd.DataFrame(data_qte)
print(df_qte)
Quantile DML QTE DML QTE lower DML QTE upper
0.10 0.10 1210.0 -163.857765 2583.857765
0.15 0.15 1230.0 485.090025 1974.909975
0.20 0.20 1211.0 499.415988 1922.584012
0.25 0.25 1000.0 308.488485 1691.511515
0.30 0.30 622.0 -98.913485 1342.913485
0.35 0.35 1031.0 254.838457 1807.161543
0.40 0.40 2006.0 1101.755910 2910.244090
0.45 0.45 3329.0 2122.065451 4535.934549
0.50 0.50 4601.0 3335.395889 5866.604111
0.55 0.55 6000.0 4336.993575 7663.006425
0.60 0.60 7040.0 5329.197711 8750.802289
0.65 0.65 9223.0 6950.717130 11495.282870
0.70 0.70 10928.0 8499.917066 13356.082934
0.75 0.75 12410.0 9534.518782 15285.481218
0.80 0.80 16590.0 12101.036945 21078.963055
0.85 0.85 19382.0 14798.973331 23965.026669
0.90 0.90 21550.0 15113.220088 27986.779912
[14]:
plt.rcParams['figure.figsize'] = 10., 7.5
fig, ax = plt.subplots()
ax.grid(visible=True)
ax.plot(df_qte['Quantile'],df_qte['DML QTE'], color='violet', label='Estimated QTE')
ax.fill_between(df_qte['Quantile'], df_qte['DML QTE lower'], df_qte['DML QTE upper'], color='violet', alpha=.3, label='Confidence Interval')
plt.legend()
plt.title('Quantile Treatment Effects', fontsize=16)
plt.xlabel('Quantile')
_ = plt.ylabel('QTE and 95%-CI')
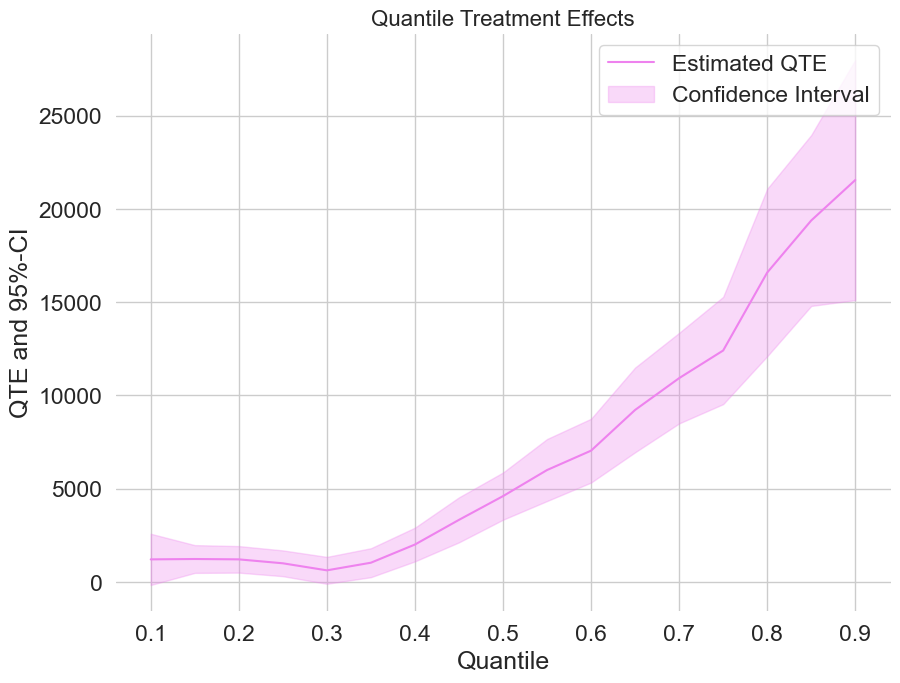
Estimating the treatment effect on the Conditional Value a Risk (CVaR)#
Similar to the evaluation of the estimation of quantile treatment effects (QTEs), we can estimate the conditional value at risk (CVaR) for given quantiles. Here, we will only focus on treatment effect estimation, but the DoubleML package also allows for estimation of potential CVaRs.
The estimation of treatment effects can be easily done by adjusting the score in the DoubleMLQTE
object to score="CVaR"
, as the estimation is based on the same nuisance elements as QTEs.
[15]:
np.random.seed(42)
dml_CVAR = dml.DoubleMLQTE(data_dml_base,
ml_g=clone(reg_learner),
ml_m=clone(class_learner),
quantiles=tau_vec,
score="CVaR",
n_folds=n_folds,
normalize_ipw=True,
trimming_rule="truncate",
trimming_threshold=1e-2)
dml_CVAR.fit(n_jobs_models=cores_used)
print(dml_CVAR)
================== DoubleMLQTE Object ==================
------------------ Fit summary ------------------
coef std err t P>|t| 2.5 % \
0.10 9073.195547 1298.264884 6.988709 2.774271e-12 6528.643133
0.15 10126.150334 1371.682269 7.382286 1.555949e-13 7437.702489
0.20 14587.388871 1485.887345 9.817291 9.485812e-23 11675.103189
0.25 16910.113415 1582.022969 10.688918 1.147015e-26 13809.405374
0.30 14744.693690 1676.606759 8.794366 1.438578e-18 11458.604825
0.35 16241.221419 1812.325090 8.961539 3.201788e-19 12689.129514
0.40 18666.064161 1970.604016 9.472255 2.738659e-21 14803.751261
0.45 12861.546294 2086.920645 6.162930 7.141098e-10 8771.256992
0.50 13642.272662 2295.693316 5.942550 2.806218e-09 9142.796444
0.55 14772.077161 2543.121399 5.808640 6.298228e-09 9787.650810
0.60 15556.468919 2849.994851 5.458420 4.803902e-08 9970.581655
0.65 16597.988780 3234.712082 5.131211 2.878847e-07 10258.069600
0.70 17576.743247 3745.384777 4.692907 2.693497e-06 10235.923977
0.75 18789.942489 4437.655422 4.234205 2.293617e-05 10092.297687
0.80 19794.747646 5476.213026 3.614678 3.007210e-04 9061.567343
0.85 19824.888804 7155.563528 2.770556 5.596069e-03 5800.242000
0.90 20055.810363 10406.538013 1.927232 5.395076e-02 -340.629346
97.5 %
0.10 11617.747961
0.15 12814.598178
0.20 17499.674552
0.25 20010.821457
0.30 18030.782555
0.35 19793.313324
0.40 22528.377060
0.45 16951.835596
0.50 18141.748880
0.55 19756.503511
0.60 21142.356183
0.65 22937.907961
0.70 24917.562518
0.75 27487.587292
0.80 30527.927950
0.85 33849.535609
0.90 40452.250073
Estimation of the corresponding (uniformly) valid confidence intervals can be done analogously to the quantile treatment effects.
[16]:
dml_CVAR.bootstrap(n_rep_boot=2000)
ci_CVAR = dml_CVAR.confint(level=0.95, joint=True)
data_cvar = {"Quantile": tau_vec, "DML CVAR": dml_CVAR.coef,
"DML CVAR lower": ci_CVAR["2.5 %"], "DML CVAR upper": ci_CVAR["97.5 %"]}
df_cvar = pd.DataFrame(data_cvar)
print(df_cvar)
Quantile DML CVAR DML CVAR lower DML CVAR upper
0.10 0.10 9073.195547 6266.876549 11879.514545
0.15 0.15 10126.150334 7161.132903 13091.167765
0.20 0.20 14587.388871 11375.506659 17799.271083
0.25 0.25 16910.113415 13490.425208 20329.801623
0.30 0.30 14744.693690 11120.553916 18368.833464
0.35 0.35 16241.221419 12323.713986 20158.728852
0.40 0.40 18666.064161 14406.422266 22925.706056
0.45 0.45 12861.546294 8350.475304 17372.617283
0.50 0.50 13642.272662 8679.920335 18604.624988
0.55 0.55 14772.077161 9274.886266 20269.268055
0.60 0.60 15556.468919 9395.942823 21716.995015
0.65 0.65 16597.988780 9605.860992 23590.116569
0.70 0.70 17576.743247 9480.749443 25672.737052
0.75 0.75 18789.942489 9197.541990 28382.342989
0.80 0.80 19794.747646 7957.409328 31632.085965
0.85 0.85 19824.888804 4357.479860 35292.297749
0.90 0.90 20055.810363 -2438.879049 42550.499776
Finally, let us take a look at the estimated treatment effects on the CVaR.
[17]:
plt.rcParams['figure.figsize'] = 10., 7.5
fig, ax = plt.subplots()
ax.grid(visible=True)
ax.plot(df_cvar['Quantile'],df_cvar['DML CVAR'], color='violet', label='Estimated CVaR Effect')
ax.fill_between(df_cvar['Quantile'], df_cvar['DML CVAR lower'], df_cvar['DML CVAR upper'], color='violet', alpha=.3, label='Confidence Interval')
plt.legend()
plt.title('Conditional Value at Risk', fontsize=16)
plt.xlabel('Quantile')
_ = plt.ylabel('CVaR Effect and 95%-CI')
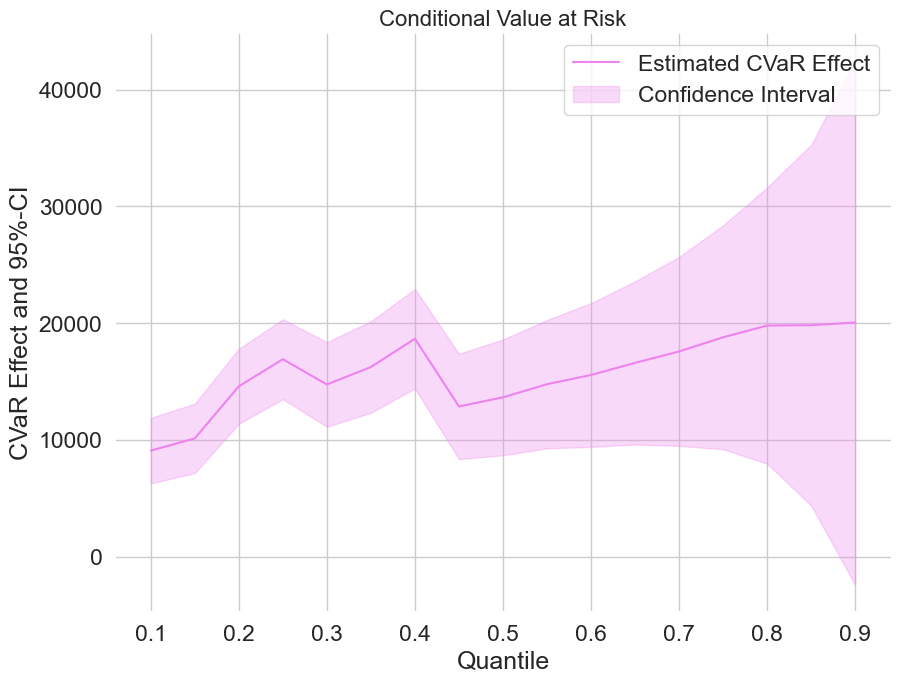
Estimating local quantile treatment effects (LQTEs)#
If we have an IIVM
model with a given instrumental variable, we are still able to identify the local quantile treatment effect (LQTE), the quantile treatment effect on compliers. For the 401(k) pension data we can use e401
as an instrument for participation p401
. To fit an DoubleML
model with an instrument, we have to change the data backend and specify the instrument.
[18]:
# Initialize DoubleMLData with an instrument
# Basic model
data_dml_base_iv = dml.DoubleMLData(data,
y_col='net_tfa',
d_cols='p401',
z_cols='e401',
x_cols=features_base)
print(data_dml_base_iv)
================== DoubleMLData Object ==================
------------------ Data summary ------------------
Outcome variable: net_tfa
Treatment variable(s): ['p401']
Covariates: ['age', 'inc', 'educ', 'fsize', 'marr', 'twoearn', 'db', 'pira', 'hown']
Instrument variable(s): ['e401']
No. Observations: 9915
------------------ DataFrame info ------------------
<class 'pandas.core.frame.DataFrame'>
RangeIndex: 9915 entries, 0 to 9914
Columns: 14 entries, nifa to hown
dtypes: float32(4), int8(10)
memory usage: 251.9 KB
The estimation of local treatment effects can be easily done by adjusting the score in the DoubleMLQTE
object to score="LPQ"
.
[19]:
np.random.seed(42)
dml_LQTE = dml.DoubleMLQTE(data_dml_base_iv,
ml_g=clone(class_learner),
ml_m=clone(class_learner),
quantiles=tau_vec,
score="LPQ",
n_folds=n_folds,
normalize_ipw=True,
trimming_rule="truncate",
trimming_threshold=1e-2)
dml_LQTE.fit(n_jobs_models=cores_used)
print(dml_LQTE)
================== DoubleMLQTE Object ==================
------------------ Fit summary ------------------
coef std err t P>|t| 2.5 % \
0.10 2610.0 487.701966 5.351629 8.716595e-08 1654.121711
0.15 1773.0 357.148790 4.964318 6.894307e-07 1073.001234
0.20 1398.0 386.526532 3.616828 2.982353e-04 640.421919
0.25 1435.0 384.956574 3.727693 1.932404e-04 680.498979
0.30 1400.0 436.977295 3.203828 1.356136e-03 543.540240
0.35 2500.0 486.877153 5.134765 2.824961e-07 1545.738315
0.40 3985.0 596.725087 6.678117 2.420316e-11 2815.440320
0.45 5175.0 739.897240 6.994214 2.667492e-12 3724.828058
0.50 7239.0 775.751013 9.331602 1.042822e-20 5718.555954
0.55 9500.0 1109.023955 8.566091 1.070574e-17 7326.352990
0.60 11750.0 1295.711518 9.068377 1.208034e-19 9210.452091
0.65 14625.0 1443.080854 10.134567 3.880880e-24 11796.613498
0.70 16984.0 1576.564577 10.772791 4.627588e-27 13893.990210
0.75 19758.0 2865.426736 6.895308 5.374821e-12 14141.866798
0.80 23856.0 2281.099670 10.458114 1.345065e-25 19385.126802
0.85 27751.0 3151.771741 8.804889 1.309823e-18 21573.640900
0.90 30645.0 4634.200110 6.612792 3.771390e-11 21562.134687
97.5 %
0.10 3565.878289
0.15 2472.998766
0.20 2155.578081
0.25 2189.501021
0.30 2256.459760
0.35 3454.261685
0.40 5154.559680
0.45 6625.171942
0.50 8759.444046
0.55 11673.647010
0.60 14289.547909
0.65 17453.386502
0.70 20074.009790
0.75 25374.133202
0.80 28326.873198
0.85 33928.359100
0.90 39727.865313
Estimation of the corresponding (uniformly) valid confidence intervals can be done analogously to the quantile treatment effects.
[20]:
dml_LQTE.bootstrap(n_rep_boot=2000)
ci_LQTE = dml_LQTE.confint(level=0.95, joint=True)
data_lqte = {"Quantile": tau_vec, "DML LQTE": dml_LQTE.coef,
"DML LQTE lower": ci_LQTE["2.5 %"], "DML LQTE upper": ci_LQTE["97.5 %"]}
df_lqte = pd.DataFrame(data_lqte)
print(df_lqte)
Quantile DML LQTE DML LQTE lower DML LQTE upper
0.10 0.10 2610.0 1255.980026 3964.019974
0.15 0.15 1773.0 781.438289 2764.561711
0.20 0.20 1398.0 324.876083 2471.123917
0.25 0.25 1435.0 366.234798 2503.765202
0.30 0.30 1400.0 186.808284 2613.191716
0.35 0.35 2500.0 1148.269977 3851.730023
0.40 0.40 3985.0 2328.296228 5641.703772
0.45 0.45 5175.0 3120.803563 7229.196437
0.50 0.50 7239.0 5085.261777 9392.738223
0.55 0.55 9500.0 6420.987220 12579.012780
0.60 0.60 11750.0 8152.681562 15347.318438
0.65 0.65 14625.0 10618.536143 18631.463857
0.70 0.70 16984.0 12606.941724 21361.058276
0.75 0.75 19758.0 11802.639345 27713.360655
0.80 0.80 23856.0 17522.922160 30189.077840
0.85 0.85 27751.0 19000.652071 36501.347929
0.90 0.90 30645.0 17778.946658 43511.053342
Finally, let us take a look at the estimated local quantile treatment effects.
[21]:
plt.rcParams['figure.figsize'] = 10., 7.5
fig, ax = plt.subplots()
ax.grid(visible=True)
ax.plot(df_lqte['Quantile'],df_lqte['DML LQTE'], color='violet', label='Estimated LQTE')
ax.fill_between(df_lqte['Quantile'], df_lqte['DML LQTE lower'], df_lqte['DML LQTE upper'], color='violet', alpha=.3, label='Confidence Interval')
plt.legend()
plt.title('Local Quantile Treatment Effect', fontsize=16)
plt.xlabel('Quantile')
_ = plt.ylabel('LQTE and 95%-CI')
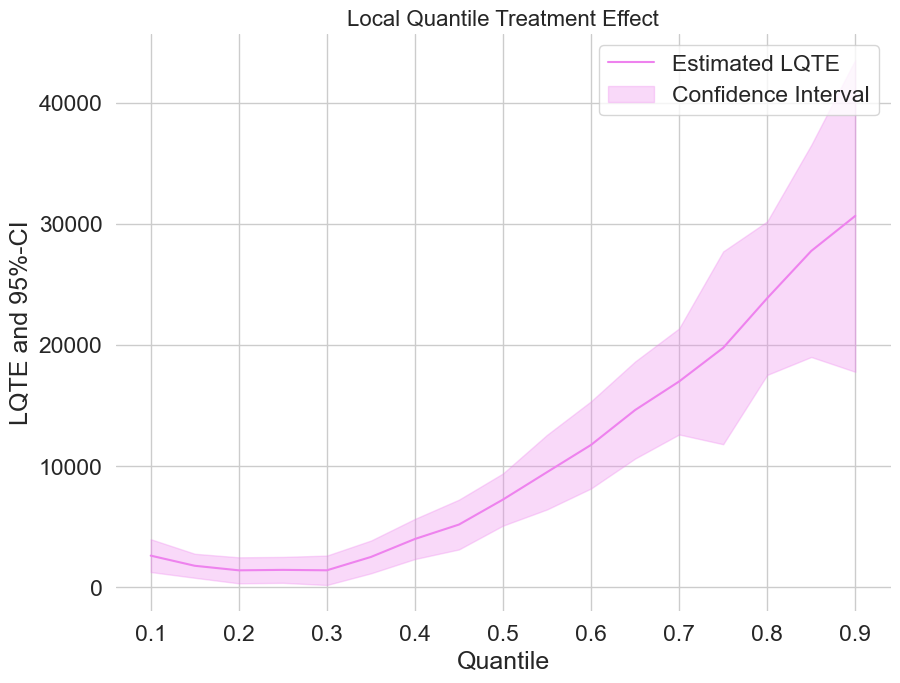