Note
-
Download Jupyter notebook:
https://docs.doubleml.org/stable/examples/py_double_ml_cate.ipynb.
Python: Conditional Average Treatment Effects (CATEs) for IRM models#
In this simple example, we illustrate how the DoubleML package can be used to estimate conditional average treatment effects with B-splines for one or two-dimensional effects in the DoubleMLIRM model.
[1]:
import numpy as np
import pandas as pd
import doubleml as dml
from doubleml.datasets import make_heterogeneous_data
Data#
We define a data generating process to create synthetic data to compare the estimates to the true effect. The data generating process is based on the Monte Carlo simulation from Oprescu et al. (2019).
The documentation of the data generating process can be found here.
One-dimensional Example#
We start with an one-dimensional effect and create our training data. In this example the true effect depends only the first covariate \(X_0\) and takes the following form
The generated dictionary also contains a callable with key treatment_effect
to calculate the true treatment effect for new observations.
[2]:
np.random.seed(42)
data_dict = make_heterogeneous_data(
n_obs=2000,
p=10,
support_size=5,
n_x=1,
binary_treatment=True,
)
treatment_effect = data_dict['treatment_effect']
data = data_dict['data']
print(data.head())
y d X_0 X_1 X_2 X_3 X_4 X_5 \
0 4.803300 1.0 0.259828 0.886086 0.895690 0.297287 0.229994 0.411304
1 5.655547 1.0 0.824350 0.396992 0.156317 0.737951 0.360475 0.671271
2 1.878402 0.0 0.988421 0.977280 0.793818 0.659423 0.577807 0.866102
3 6.941440 1.0 0.427486 0.330285 0.564232 0.850575 0.201528 0.934433
4 1.703049 1.0 0.016200 0.818380 0.040139 0.889913 0.991963 0.294067
X_6 X_7 X_8 X_9
0 0.240532 0.672384 0.826065 0.673092
1 0.270644 0.081230 0.992582 0.156202
2 0.289440 0.467681 0.619390 0.411190
3 0.689088 0.823273 0.556191 0.779517
4 0.210319 0.765363 0.253026 0.865562
First, define the DoubleMLData
object.
[3]:
data_dml_base = dml.DoubleMLData(
data,
y_col='y',
d_cols='d'
)
Next, define the learners for the nuisance functions and fit the IRM Model. Remark that linear learners would usually be optimal due to the data generating process.
[4]:
# First stage estimation
from sklearn.ensemble import RandomForestClassifier, RandomForestRegressor
randomForest_reg = RandomForestRegressor(n_estimators=500)
randomForest_class = RandomForestClassifier(n_estimators=500)
np.random.seed(42)
dml_irm = dml.DoubleMLIRM(data_dml_base,
ml_g=randomForest_reg,
ml_m=randomForest_class,
trimming_threshold=0.05,
n_folds=5)
print("Training IRM Model")
dml_irm.fit()
print(dml_irm.summary)
Training IRM Model
coef std err t P>|t| 2.5 % 97.5 %
d 4.475569 0.0408 109.695045 0.0 4.395603 4.555536
To estimate the CATE, we rely on the best-linear-predictor of the linear score as in Semenova et al. (2021) To approximate the target function \(\theta_0(x)\) with a linear form, we have to define a data frame of basis functions. Here, we rely on patsy to construct a suitable basis of B-splines.
[5]:
import patsy
design_matrix = patsy.dmatrix("bs(x, df=5, degree=2)", {"x": data["X_0"]})
spline_basis = pd.DataFrame(design_matrix)
To estimate the parameters to calculate the CATE estimate call the cate()
method and supply the dataframe of basis elements.
[6]:
cate = dml_irm.cate(spline_basis)
print(cate)
================== DoubleMLBLP Object ==================
------------------ Fit summary ------------------
coef std err t P>|t| [0.025 0.975]
0 0.691423 0.143534 4.817119 1.456458e-06 0.410100 0.972745
1 2.303007 0.247977 9.287196 1.584057e-20 1.816982 2.789032
2 4.904315 0.161269 30.410682 3.968258e-203 4.588233 5.220398
3 4.755688 0.194092 24.502205 1.399343e-132 4.375274 5.136102
4 3.745881 0.195781 19.132982 1.341755e-81 3.362157 4.129606
5 4.314341 0.200049 21.566388 3.716013e-103 3.922251 4.706430
To obtain the confidence intervals for the CATE, we have to call the confint()
method and a supply a dataframe of basis elements. This could be the same basis as for fitting the CATE model or a new basis to e.g. evaluate the CATE model on a grid. Here, we will evaluate the CATE on a grid from 0.1 to 0.9 to plot the final results. Further, we construct uniform confidence intervals by setting the option joint
and providing a number of bootstrap repetitions n_rep_boot
.
[7]:
new_data = {"x": np.linspace(0.1, 0.9, 100)}
spline_grid = pd.DataFrame(patsy.build_design_matrices([design_matrix.design_info], new_data)[0])
df_cate = cate.confint(spline_grid, level=0.95, joint=True, n_rep_boot=2000)
print(df_cate)
2.5 % effect 97.5 %
0 2.070552 2.333655 2.596758
1 2.185984 2.453279 2.720573
2 2.298076 2.570936 2.843796
3 2.407558 2.686627 2.965696
4 2.515031 2.800351 3.085671
.. ... ... ...
95 4.417640 4.704814 4.991988
96 4.424292 4.705354 4.986417
97 4.433750 4.708235 4.982720
98 4.445476 4.713457 4.981438
99 4.458784 4.721018 4.983253
[100 rows x 3 columns]
Finally, we can plot our results and compare them with the true effect.
[8]:
from matplotlib import pyplot as plt
plt.rcParams['figure.figsize'] = 10., 7.5
df_cate['x'] = new_data['x']
df_cate['true_effect'] = treatment_effect(new_data["x"].reshape(-1, 1))
fig, ax = plt.subplots()
ax.plot(df_cate['x'],df_cate['effect'], label='Estimated Effect')
ax.plot(df_cate['x'],df_cate['true_effect'], color="green", label='True Effect')
ax.fill_between(df_cate['x'], df_cate['2.5 %'], df_cate['97.5 %'], color='b', alpha=.3, label='Confidence Interval')
plt.legend()
plt.title('CATE')
plt.xlabel('x')
_ = plt.ylabel('Effect and 95%-CI')
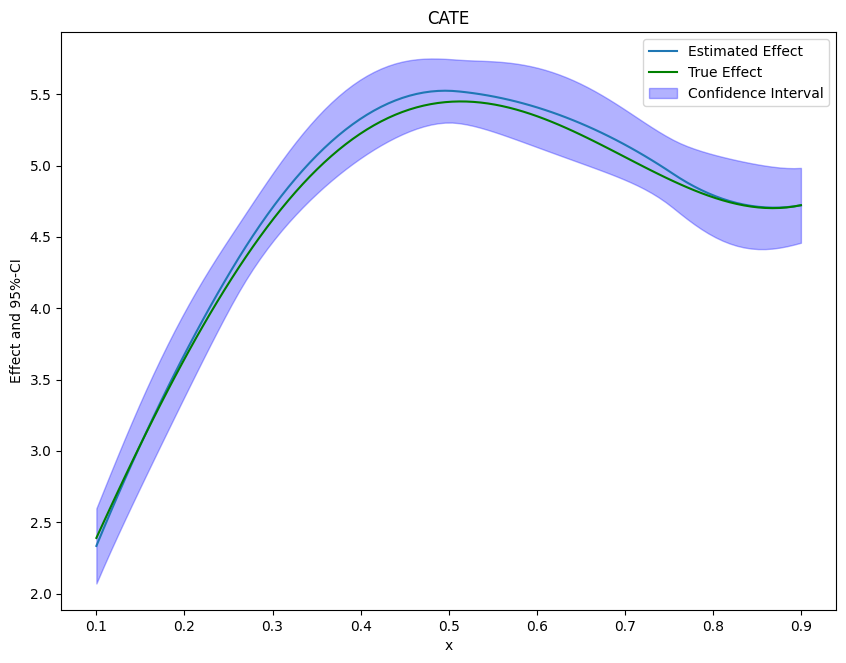
If the effect is not one-dimensional, the estimate still corresponds to the projection of the true effect on the basis functions.
Two-Dimensional Example#
It is also possible to estimate multi-dimensional conditional effects. We will use a similar data generating process but now the effect depends on the first two covariates \(X_0\) and \(X_1\) and takes the following form
With the argument n_x=2
we can specify set the effect to be two-dimensional.
[9]:
np.random.seed(42)
data_dict = make_heterogeneous_data(
n_obs=5000,
p=10,
support_size=5,
n_x=2,
binary_treatment=True,
)
treatment_effect = data_dict['treatment_effect']
data = data_dict['data']
print(data.head())
y d X_0 X_1 X_2 X_3 X_4 X_5 \
0 1.286203 1.0 0.014080 0.006958 0.240127 0.100807 0.260211 0.177043
1 0.416899 1.0 0.152148 0.912230 0.892796 0.653901 0.672234 0.005339
2 2.087634 1.0 0.344787 0.893649 0.291517 0.562712 0.099731 0.921956
3 7.508433 1.0 0.619351 0.232134 0.000943 0.757151 0.985207 0.809913
4 0.567695 0.0 0.477130 0.447624 0.775191 0.526769 0.316717 0.258158
X_6 X_7 X_8 X_9
0 0.028520 0.909304 0.008223 0.736082
1 0.984872 0.877833 0.895106 0.659245
2 0.140770 0.224897 0.558134 0.764093
3 0.460207 0.903767 0.409848 0.524934
4 0.037747 0.583195 0.229961 0.148134
As univariate example estimate the IRM Model.
[10]:
data_dml_base = dml.DoubleMLData(
data,
y_col='y',
d_cols='d'
)
[11]:
# First stage estimation
from sklearn.ensemble import RandomForestClassifier, RandomForestRegressor
randomForest_reg = RandomForestRegressor(n_estimators=500)
randomForest_class = RandomForestClassifier(n_estimators=500)
np.random.seed(42)
dml_irm = dml.DoubleMLIRM(data_dml_base,
ml_g=randomForest_reg,
ml_m=randomForest_class,
trimming_threshold=0.05,
n_folds=5)
print("Training IRM Model")
dml_irm.fit()
print(dml_irm.summary)
Training IRM Model
coef std err t P>|t| 2.5 % 97.5 %
d 4.547039 0.038845 117.056745 0.0 4.470904 4.623173
As above, we will rely on the patsy package to construct the basis elements. In the two-dimensional case, we will construct a tensor product of B-splines (for more information see here).
[12]:
design_matrix = patsy.dmatrix("te(bs(x_0, df=7, degree=3), bs(x_1, df=7, degree=3))", {"x_0": data["X_0"], "x_1": data["X_1"]})
spline_basis = pd.DataFrame(design_matrix)
cate = dml_irm.cate(spline_basis)
print(cate)
================== DoubleMLBLP Object ==================
------------------ Fit summary ------------------
coef std err t P>|t| [0.025 0.975]
0 2.805774 0.142119 19.742411 9.322186e-87 2.527226 3.084323
1 -1.115972 0.830442 -1.343828 1.790039e-01 -2.743609 0.511665
2 0.462979 0.786237 0.588854 5.559592e-01 -1.078017 2.003975
3 2.308774 0.725061 3.184247 1.451312e-03 0.887680 3.729867
4 0.575810 0.739063 0.779108 4.359161e-01 -0.872727 2.024346
5 -3.338603 1.003944 -3.325486 8.826467e-04 -5.306297 -1.370908
6 -4.994377 1.104849 -4.520415 6.171848e-06 -7.159841 -2.828912
7 -6.404411 0.950158 -6.740367 1.579875e-11 -8.266686 -4.542136
8 -2.644985 0.809125 -3.268943 1.079500e-03 -4.230842 -1.059128
9 3.753393 0.855035 4.389755 1.134784e-05 2.077555 5.429230
10 -0.018508 0.725919 -0.025496 9.796596e-01 -1.441282 1.404267
11 1.577813 0.797086 1.979475 4.776254e-02 0.015552 3.140073
12 -1.070751 1.092935 -0.979702 3.272332e-01 -3.212863 1.071362
13 -2.291008 1.193253 -1.919969 5.486178e-02 -4.629740 0.047724
14 -2.607264 0.939458 -2.775285 5.515338e-03 -4.448569 -0.765960
15 0.241678 0.732137 0.330100 7.413247e-01 -1.193285 1.676641
16 1.085395 0.730809 1.485197 1.374917e-01 -0.346964 2.517753
17 3.742375 0.648355 5.772104 7.828778e-09 2.471622 5.013128
18 1.384928 0.677123 2.045313 4.082400e-02 0.057792 2.712064
19 -1.506050 0.912903 -1.649738 9.899662e-02 -3.295307 0.283207
20 -2.315310 1.013712 -2.283992 2.237200e-02 -4.302149 -0.328471
21 -2.806554 0.753323 -3.725565 1.948785e-04 -4.283041 -1.330068
22 1.945881 0.723657 2.688956 7.167581e-03 0.527540 3.364221
23 2.189248 0.754469 2.901705 3.711383e-03 0.710515 3.667981
24 4.374862 0.672511 6.505264 7.755701e-11 3.056764 5.692959
25 2.134542 0.649514 3.286371 1.014873e-03 0.861519 3.407565
26 1.709596 0.904396 1.890318 5.871545e-02 -0.062988 3.482179
27 -1.233029 0.981715 -1.255995 2.091179e-01 -3.157154 0.691097
28 -1.378588 0.847962 -1.625767 1.039991e-01 -3.040562 0.283386
29 4.060417 0.953704 4.257523 2.067046e-05 2.191192 5.929643
30 4.063700 0.885956 4.586794 4.501047e-06 2.327257 5.800143
31 6.076596 0.810322 7.498992 6.431061e-14 4.488394 7.664797
32 3.840673 0.778400 4.934058 8.053849e-07 2.315036 5.366310
33 2.584928 1.058595 2.441849 1.461227e-02 0.510121 4.659735
34 -1.847555 1.300031 -1.421163 1.552694e-01 -4.395569 0.700458
35 -0.429705 1.107413 -0.388026 6.979971e-01 -2.600195 1.740785
36 7.039036 1.009255 6.974487 3.069882e-12 5.060933 9.017140
37 5.187664 0.990903 5.235291 1.647254e-07 3.245531 7.129798
38 7.151063 0.831278 8.602492 7.800326e-18 5.521788 8.780338
39 6.733644 0.916930 7.343685 2.077923e-13 4.936494 8.530793
40 2.381603 1.177740 2.022181 4.315769e-02 0.073275 4.689932
41 4.440747 1.294449 3.430608 6.022295e-04 1.903674 6.977820
42 1.732067 1.095654 1.580853 1.139117e-01 -0.415375 3.879509
43 10.068514 1.002277 10.045638 9.602386e-24 8.104087 12.032941
44 3.734635 1.100715 3.392917 6.915260e-04 1.577273 5.891997
45 9.197920 0.697693 13.183339 1.094378e-39 7.830467 10.565373
46 5.367181 0.695928 7.712268 1.236015e-14 4.003187 6.731174
47 5.925660 0.816373 7.258522 3.913415e-13 4.325599 7.525722
48 1.301737 0.988541 1.316826 1.878968e-01 -0.635768 3.239243
49 1.237341 0.897451 1.378727 1.679789e-01 -0.521632 2.996313
Finally, we create a new grid to evaluate and plot the effects.
[13]:
grid_size = 100
x_0 = np.linspace(0.1, 0.9, grid_size)
x_1 = np.linspace(0.1, 0.9, grid_size)
x_0, x_1 = np.meshgrid(x_0, x_1)
new_data = {"x_0": x_0.ravel(), "x_1": x_1.ravel()}
[14]:
spline_grid = pd.DataFrame(patsy.build_design_matrices([design_matrix.design_info], new_data)[0])
df_cate = cate.confint(spline_grid, joint=True, n_rep_boot=2000)
print(df_cate)
2.5 % effect 97.5 %
0 1.671690 2.379626 3.087561
1 1.681521 2.366950 3.052380
2 1.698509 2.357170 3.015831
3 1.720559 2.350208 2.979857
4 1.745444 2.345989 2.946533
... ... ... ...
9995 3.716387 4.506644 5.296901
9996 3.831741 4.661388 5.491034
9997 3.939250 4.811696 5.684142
9998 4.041925 4.955701 5.869477
9999 4.142382 5.091535 6.040688
[10000 rows x 3 columns]
[15]:
import plotly.graph_objects as go
grid_array = np.array(list(zip(x_0.ravel(), x_1.ravel())))
true_effect = treatment_effect(grid_array).reshape(x_0.shape)
effect = np.asarray(df_cate['effect']).reshape(x_0.shape)
lower_bound = np.asarray(df_cate['2.5 %']).reshape(x_0.shape)
upper_bound = np.asarray(df_cate['97.5 %']).reshape(x_0.shape)
fig = go.Figure(data=[
go.Surface(x=x_0,
y=x_1,
z=true_effect),
go.Surface(x=x_0,
y=x_1,
z=upper_bound, showscale=False, opacity=0.4,colorscale='purp'),
go.Surface(x=x_0,
y=x_1,
z=lower_bound, showscale=False, opacity=0.4,colorscale='purp'),
])
fig.update_traces(contours_z=dict(show=True, usecolormap=True,
highlightcolor="limegreen", project_z=True))
fig.update_layout(scene = dict(
xaxis_title='X_0',
yaxis_title='X_1',
zaxis_title='Effect'),
width=700,
margin=dict(r=20, b=10, l=10, t=10))
fig.show()